Skip to content
Read the latest news stories about Mailman faculty, research, and events.

Departments
We integrate an innovative skills-based curriculum, research collaborations, and hands-on field experience to prepare students.
Learn more about our research centers, which focus on critical issues in public health.
Our Faculty
Meet the faculty of the Mailman School of Public Health.
Become a Student
Life and community, how to apply.
Learn how to apply to the Mailman School of Public Health.
Content Analysis
Content analysis is a research tool used to determine the presence of certain words, themes, or concepts within some given qualitative data (i.e. text). Using content analysis, researchers can quantify and analyze the presence, meanings, and relationships of such certain words, themes, or concepts. As an example, researchers can evaluate language used within a news article to search for bias or partiality. Researchers can then make inferences about the messages within the texts, the writer(s), the audience, and even the culture and time of surrounding the text.
Description
Sources of data could be from interviews, open-ended questions, field research notes, conversations, or literally any occurrence of communicative language (such as books, essays, discussions, newspaper headlines, speeches, media, historical documents). A single study may analyze various forms of text in its analysis. To analyze the text using content analysis, the text must be coded, or broken down, into manageable code categories for analysis (i.e. “codes”). Once the text is coded into code categories, the codes can then be further categorized into “code categories” to summarize data even further.
Three different definitions of content analysis are provided below.
Definition 1: “Any technique for making inferences by systematically and objectively identifying special characteristics of messages.” (from Holsti, 1968)
Definition 2: “An interpretive and naturalistic approach. It is both observational and narrative in nature and relies less on the experimental elements normally associated with scientific research (reliability, validity, and generalizability) (from Ethnography, Observational Research, and Narrative Inquiry, 1994-2012).
Definition 3: “A research technique for the objective, systematic and quantitative description of the manifest content of communication.” (from Berelson, 1952)
Uses of Content Analysis
Identify the intentions, focus or communication trends of an individual, group or institution
Describe attitudinal and behavioral responses to communications
Determine the psychological or emotional state of persons or groups
Reveal international differences in communication content
Reveal patterns in communication content
Pre-test and improve an intervention or survey prior to launch
Analyze focus group interviews and open-ended questions to complement quantitative data
Types of Content Analysis
There are two general types of content analysis: conceptual analysis and relational analysis. Conceptual analysis determines the existence and frequency of concepts in a text. Relational analysis develops the conceptual analysis further by examining the relationships among concepts in a text. Each type of analysis may lead to different results, conclusions, interpretations and meanings.
Conceptual Analysis
Typically people think of conceptual analysis when they think of content analysis. In conceptual analysis, a concept is chosen for examination and the analysis involves quantifying and counting its presence. The main goal is to examine the occurrence of selected terms in the data. Terms may be explicit or implicit. Explicit terms are easy to identify. Coding of implicit terms is more complicated: you need to decide the level of implication and base judgments on subjectivity (an issue for reliability and validity). Therefore, coding of implicit terms involves using a dictionary or contextual translation rules or both.
To begin a conceptual content analysis, first identify the research question and choose a sample or samples for analysis. Next, the text must be coded into manageable content categories. This is basically a process of selective reduction. By reducing the text to categories, the researcher can focus on and code for specific words or patterns that inform the research question.
General steps for conducting a conceptual content analysis:
1. Decide the level of analysis: word, word sense, phrase, sentence, themes
2. Decide how many concepts to code for: develop a pre-defined or interactive set of categories or concepts. Decide either: A. to allow flexibility to add categories through the coding process, or B. to stick with the pre-defined set of categories.
Option A allows for the introduction and analysis of new and important material that could have significant implications to one’s research question.
Option B allows the researcher to stay focused and examine the data for specific concepts.
3. Decide whether to code for existence or frequency of a concept. The decision changes the coding process.
When coding for the existence of a concept, the researcher would count a concept only once if it appeared at least once in the data and no matter how many times it appeared.
When coding for the frequency of a concept, the researcher would count the number of times a concept appears in a text.
4. Decide on how you will distinguish among concepts:
Should text be coded exactly as they appear or coded as the same when they appear in different forms? For example, “dangerous” vs. “dangerousness”. The point here is to create coding rules so that these word segments are transparently categorized in a logical fashion. The rules could make all of these word segments fall into the same category, or perhaps the rules can be formulated so that the researcher can distinguish these word segments into separate codes.
What level of implication is to be allowed? Words that imply the concept or words that explicitly state the concept? For example, “dangerous” vs. “the person is scary” vs. “that person could cause harm to me”. These word segments may not merit separate categories, due the implicit meaning of “dangerous”.
5. Develop rules for coding your texts. After decisions of steps 1-4 are complete, a researcher can begin developing rules for translation of text into codes. This will keep the coding process organized and consistent. The researcher can code for exactly what he/she wants to code. Validity of the coding process is ensured when the researcher is consistent and coherent in their codes, meaning that they follow their translation rules. In content analysis, obeying by the translation rules is equivalent to validity.
6. Decide what to do with irrelevant information: should this be ignored (e.g. common English words like “the” and “and”), or used to reexamine the coding scheme in the case that it would add to the outcome of coding?
7. Code the text: This can be done by hand or by using software. By using software, researchers can input categories and have coding done automatically, quickly and efficiently, by the software program. When coding is done by hand, a researcher can recognize errors far more easily (e.g. typos, misspelling). If using computer coding, text could be cleaned of errors to include all available data. This decision of hand vs. computer coding is most relevant for implicit information where category preparation is essential for accurate coding.
8. Analyze your results: Draw conclusions and generalizations where possible. Determine what to do with irrelevant, unwanted, or unused text: reexamine, ignore, or reassess the coding scheme. Interpret results carefully as conceptual content analysis can only quantify the information. Typically, general trends and patterns can be identified.
Relational Analysis
Relational analysis begins like conceptual analysis, where a concept is chosen for examination. However, the analysis involves exploring the relationships between concepts. Individual concepts are viewed as having no inherent meaning and rather the meaning is a product of the relationships among concepts.
To begin a relational content analysis, first identify a research question and choose a sample or samples for analysis. The research question must be focused so the concept types are not open to interpretation and can be summarized. Next, select text for analysis. Select text for analysis carefully by balancing having enough information for a thorough analysis so results are not limited with having information that is too extensive so that the coding process becomes too arduous and heavy to supply meaningful and worthwhile results.
There are three subcategories of relational analysis to choose from prior to going on to the general steps.
Affect extraction: an emotional evaluation of concepts explicit in a text. A challenge to this method is that emotions can vary across time, populations, and space. However, it could be effective at capturing the emotional and psychological state of the speaker or writer of the text.
Proximity analysis: an evaluation of the co-occurrence of explicit concepts in the text. Text is defined as a string of words called a “window” that is scanned for the co-occurrence of concepts. The result is the creation of a “concept matrix”, or a group of interrelated co-occurring concepts that would suggest an overall meaning.
Cognitive mapping: a visualization technique for either affect extraction or proximity analysis. Cognitive mapping attempts to create a model of the overall meaning of the text such as a graphic map that represents the relationships between concepts.
General steps for conducting a relational content analysis:
1. Determine the type of analysis: Once the sample has been selected, the researcher needs to determine what types of relationships to examine and the level of analysis: word, word sense, phrase, sentence, themes. 2. Reduce the text to categories and code for words or patterns. A researcher can code for existence of meanings or words. 3. Explore the relationship between concepts: once the words are coded, the text can be analyzed for the following:
Strength of relationship: degree to which two or more concepts are related.
Sign of relationship: are concepts positively or negatively related to each other?
Direction of relationship: the types of relationship that categories exhibit. For example, “X implies Y” or “X occurs before Y” or “if X then Y” or if X is the primary motivator of Y.
4. Code the relationships: a difference between conceptual and relational analysis is that the statements or relationships between concepts are coded. 5. Perform statistical analyses: explore differences or look for relationships among the identified variables during coding. 6. Map out representations: such as decision mapping and mental models.
Reliability and Validity
Reliability : Because of the human nature of researchers, coding errors can never be eliminated but only minimized. Generally, 80% is an acceptable margin for reliability. Three criteria comprise the reliability of a content analysis:
Stability: the tendency for coders to consistently re-code the same data in the same way over a period of time.
Reproducibility: tendency for a group of coders to classify categories membership in the same way.
Accuracy: extent to which the classification of text corresponds to a standard or norm statistically.
Validity : Three criteria comprise the validity of a content analysis:
Closeness of categories: this can be achieved by utilizing multiple classifiers to arrive at an agreed upon definition of each specific category. Using multiple classifiers, a concept category that may be an explicit variable can be broadened to include synonyms or implicit variables.
Conclusions: What level of implication is allowable? Do conclusions correctly follow the data? Are results explainable by other phenomena? This becomes especially problematic when using computer software for analysis and distinguishing between synonyms. For example, the word “mine,” variously denotes a personal pronoun, an explosive device, and a deep hole in the ground from which ore is extracted. Software can obtain an accurate count of that word’s occurrence and frequency, but not be able to produce an accurate accounting of the meaning inherent in each particular usage. This problem could throw off one’s results and make any conclusion invalid.
Generalizability of the results to a theory: dependent on the clear definitions of concept categories, how they are determined and how reliable they are at measuring the idea one is seeking to measure. Generalizability parallels reliability as much of it depends on the three criteria for reliability.
Advantages of Content Analysis
Directly examines communication using text
Allows for both qualitative and quantitative analysis
Provides valuable historical and cultural insights over time
Allows a closeness to data
Coded form of the text can be statistically analyzed
Unobtrusive means of analyzing interactions
Provides insight into complex models of human thought and language use
When done well, is considered a relatively “exact” research method
Content analysis is a readily-understood and an inexpensive research method
A more powerful tool when combined with other research methods such as interviews, observation, and use of archival records. It is very useful for analyzing historical material, especially for documenting trends over time.
Disadvantages of Content Analysis
Can be extremely time consuming
Is subject to increased error, particularly when relational analysis is used to attain a higher level of interpretation
Is often devoid of theoretical base, or attempts too liberally to draw meaningful inferences about the relationships and impacts implied in a study
Is inherently reductive, particularly when dealing with complex texts
Tends too often to simply consist of word counts
Often disregards the context that produced the text, as well as the state of things after the text is produced
Can be difficult to automate or computerize
Textbooks & Chapters
Berelson, Bernard. Content Analysis in Communication Research.New York: Free Press, 1952.
Busha, Charles H. and Stephen P. Harter. Research Methods in Librarianship: Techniques and Interpretation.New York: Academic Press, 1980.
de Sola Pool, Ithiel. Trends in Content Analysis. Urbana: University of Illinois Press, 1959.
Krippendorff, Klaus. Content Analysis: An Introduction to its Methodology. Beverly Hills: Sage Publications, 1980.
Fielding, NG & Lee, RM. Using Computers in Qualitative Research. SAGE Publications, 1991. (Refer to Chapter by Seidel, J. ‘Method and Madness in the Application of Computer Technology to Qualitative Data Analysis’.)
Methodological Articles
Hsieh HF & Shannon SE. (2005). Three Approaches to Qualitative Content Analysis.Qualitative Health Research. 15(9): 1277-1288.
Elo S, Kaarianinen M, Kanste O, Polkki R, Utriainen K, & Kyngas H. (2014). Qualitative Content Analysis: A focus on trustworthiness. Sage Open. 4:1-10.
Application Articles
Abroms LC, Padmanabhan N, Thaweethai L, & Phillips T. (2011). iPhone Apps for Smoking Cessation: A content analysis. American Journal of Preventive Medicine. 40(3):279-285.
Ullstrom S. Sachs MA, Hansson J, Ovretveit J, & Brommels M. (2014). Suffering in Silence: a qualitative study of second victims of adverse events. British Medical Journal, Quality & Safety Issue. 23:325-331.
Owen P. (2012).Portrayals of Schizophrenia by Entertainment Media: A Content Analysis of Contemporary Movies. Psychiatric Services. 63:655-659.
Choosing whether to conduct a content analysis by hand or by using computer software can be difficult. Refer to ‘Method and Madness in the Application of Computer Technology to Qualitative Data Analysis’ listed above in “Textbooks and Chapters” for a discussion of the issue.
QSR NVivo: http://www.qsrinternational.com/products.aspx
Atlas.ti: http://www.atlasti.com/webinars.html
R- RQDA package: http://rqda.r-forge.r-project.org/
Rolly Constable, Marla Cowell, Sarita Zornek Crawford, David Golden, Jake Hartvigsen, Kathryn Morgan, Anne Mudgett, Kris Parrish, Laura Thomas, Erika Yolanda Thompson, Rosie Turner, and Mike Palmquist. (1994-2012). Ethnography, Observational Research, and Narrative Inquiry. Writing@CSU. Colorado State University. Available at: https://writing.colostate.edu/guides/guide.cfm?guideid=63 .
As an introduction to Content Analysis by Michael Palmquist, this is the main resource on Content Analysis on the Web. It is comprehensive, yet succinct. It includes examples and an annotated bibliography. The information contained in the narrative above draws heavily from and summarizes Michael Palmquist’s excellent resource on Content Analysis but was streamlined for the purpose of doctoral students and junior researchers in epidemiology.
At Columbia University Mailman School of Public Health, more detailed training is available through the Department of Sociomedical Sciences- P8785 Qualitative Research Methods.
Join the Conversation
Have a question about methods? Join us on Facebook

What Is Qualitative Content Analysis?
Qca explained simply (with examples).
By: Jenna Crosley (PhD). Reviewed by: Dr Eunice Rautenbach (DTech) | February 2021
If you’re in the process of preparing for your dissertation, thesis or research project, you’ve probably encountered the term “ qualitative content analysis ” – it’s quite a mouthful. If you’ve landed on this post, you’re probably a bit confused about it. Well, the good news is that you’ve come to the right place…
Overview: Qualitative Content Analysis
- What (exactly) is qualitative content analysis
- The two main types of content analysis
- When to use content analysis
- How to conduct content analysis (the process)
- The advantages and disadvantages of content analysis
1. What is content analysis?
Content analysis is a qualitative analysis method that focuses on recorded human artefacts such as manuscripts, voice recordings and journals. Content analysis investigates these written, spoken and visual artefacts without explicitly extracting data from participants – this is called unobtrusive research.
In other words, with content analysis, you don’t necessarily need to interact with participants (although you can if necessary); you can simply analyse the data that they have already produced. With this type of analysis, you can analyse data such as text messages, books, Facebook posts, videos, and audio (just to mention a few).
The basics – explicit and implicit content
When working with content analysis, explicit and implicit content will play a role. Explicit data is transparent and easy to identify, while implicit data is that which requires some form of interpretation and is often of a subjective nature. Sounds a bit fluffy? Here’s an example:
Joe: Hi there, what can I help you with?
Lauren: I recently adopted a puppy and I’m worried that I’m not feeding him the right food. Could you please advise me on what I should be feeding?
Joe: Sure, just follow me and I’ll show you. Do you have any other pets?
Lauren: Only one, and it tweets a lot!
In this exchange, the explicit data indicates that Joe is helping Lauren to find the right puppy food. Lauren asks Joe whether she has any pets aside from her puppy. This data is explicit because it requires no interpretation.
On the other hand, implicit data , in this case, includes the fact that the speakers are in a pet store. This information is not clearly stated but can be inferred from the conversation, where Joe is helping Lauren to choose pet food. An additional piece of implicit data is that Lauren likely has some type of bird as a pet. This can be inferred from the way that Lauren states that her pet “tweets”.
As you can see, explicit and implicit data both play a role in human interaction and are an important part of your analysis. However, it’s important to differentiate between these two types of data when you’re undertaking content analysis. Interpreting implicit data can be rather subjective as conclusions are based on the researcher’s interpretation. This can introduce an element of bias , which risks skewing your results.
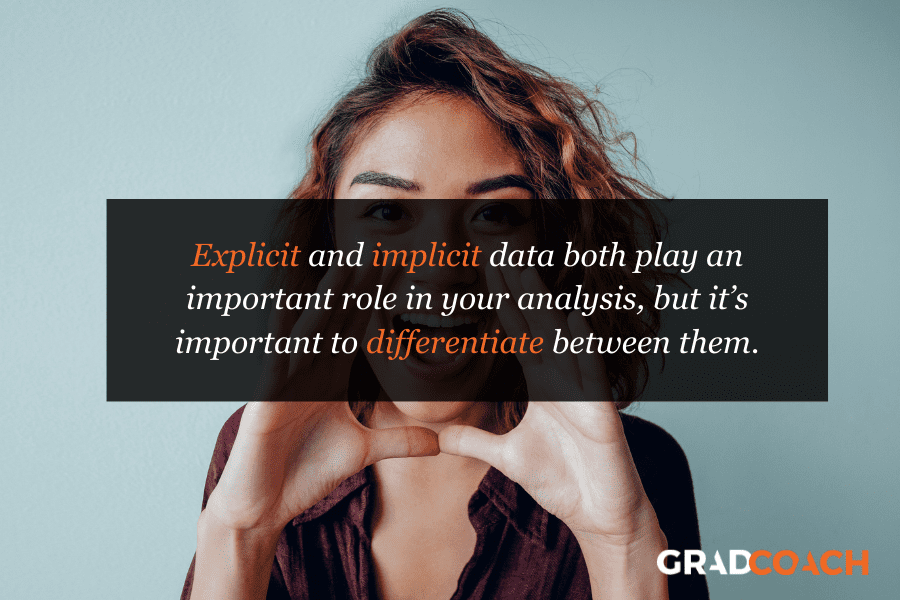
2. The two types of content analysis
Now that you understand the difference between implicit and explicit data, let’s move on to the two general types of content analysis : conceptual and relational content analysis. Importantly, while conceptual and relational content analysis both follow similar steps initially, the aims and outcomes of each are different.
Conceptual analysis focuses on the number of times a concept occurs in a set of data and is generally focused on explicit data. For example, if you were to have the following conversation:
Marie: She told me that she has three cats.
Jean: What are her cats’ names?
Marie: I think the first one is Bella, the second one is Mia, and… I can’t remember the third cat’s name.
In this data, you can see that the word “cat” has been used three times. Through conceptual content analysis, you can deduce that cats are the central topic of the conversation. You can also perform a frequency analysis , where you assess the term’s frequency in the data. For example, in the exchange above, the word “cat” makes up 9% of the data. In other words, conceptual analysis brings a little bit of quantitative analysis into your qualitative analysis.
As you can see, the above data is without interpretation and focuses on explicit data . Relational content analysis, on the other hand, takes a more holistic view by focusing more on implicit data in terms of context, surrounding words and relationships.
There are three types of relational analysis:
- Affect extraction
- Proximity analysis
- Cognitive mapping
Affect extraction is when you assess concepts according to emotional attributes. These emotions are typically mapped on scales, such as a Likert scale or a rating scale ranging from 1 to 5, where 1 is “very sad” and 5 is “very happy”.
If participants are talking about their achievements, they are likely to be given a score of 4 or 5, depending on how good they feel about it. If a participant is describing a traumatic event, they are likely to have a much lower score, either 1 or 2.
Proximity analysis identifies explicit terms (such as those found in a conceptual analysis) and the patterns in terms of how they co-occur in a text. In other words, proximity analysis investigates the relationship between terms and aims to group these to extract themes and develop meaning.
Proximity analysis is typically utilised when you’re looking for hard facts rather than emotional, cultural, or contextual factors. For example, if you were to analyse a political speech, you may want to focus only on what has been said, rather than implications or hidden meanings. To do this, you would make use of explicit data, discounting any underlying meanings and implications of the speech.
Lastly, there’s cognitive mapping, which can be used in addition to, or along with, proximity analysis. Cognitive mapping involves taking different texts and comparing them in a visual format – i.e. a cognitive map. Typically, you’d use cognitive mapping in studies that assess changes in terms, definitions, and meanings over time. It can also serve as a way to visualise affect extraction or proximity analysis and is often presented in a form such as a graphic map.
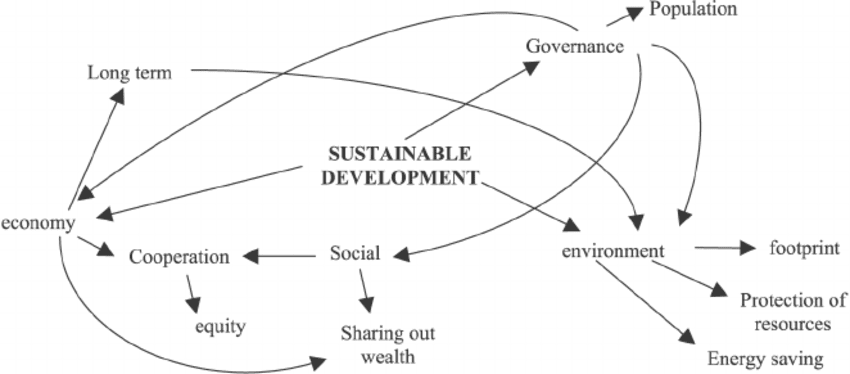
To recap on the essentials, content analysis is a qualitative analysis method that focuses on recorded human artefacts . It involves both conceptual analysis (which is more numbers-based) and relational analysis (which focuses on the relationships between concepts and how they’re connected).
Need a helping hand?
3. When should you use content analysis?
Content analysis is a useful tool that provides insight into trends of communication . For example, you could use a discussion forum as the basis of your analysis and look at the types of things the members talk about as well as how they use language to express themselves. Content analysis is flexible in that it can be applied to the individual, group, and institutional level.
Content analysis is typically used in studies where the aim is to better understand factors such as behaviours, attitudes, values, emotions, and opinions . For example, you could use content analysis to investigate an issue in society, such as miscommunication between cultures. In this example, you could compare patterns of communication in participants from different cultures, which will allow you to create strategies for avoiding misunderstandings in intercultural interactions.
Another example could include conducting content analysis on a publication such as a book. Here you could gather data on the themes, topics, language use and opinions reflected in the text to draw conclusions regarding the political (such as conservative or liberal) leanings of the publication.
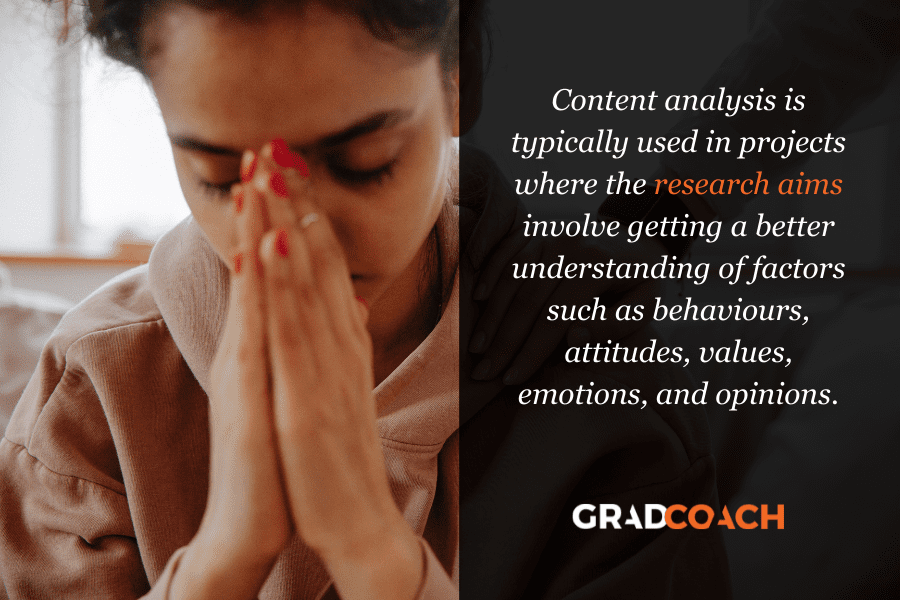
4. How to conduct a qualitative content analysis
Conceptual and relational content analysis differ in terms of their exact process ; however, there are some similarities. Let’s have a look at these first – i.e., the generic process:
- Recap on your research questions
- Undertake bracketing to identify biases
- Operationalise your variables and develop a coding scheme
- Code the data and undertake your analysis
Step 1 – Recap on your research questions
It’s always useful to begin a project with research questions , or at least with an idea of what you are looking for. In fact, if you’ve spent time reading this blog, you’ll know that it’s useful to recap on your research questions, aims and objectives when undertaking pretty much any research activity. In the context of content analysis, it’s difficult to know what needs to be coded and what doesn’t, without a clear view of the research questions.
For example, if you were to code a conversation focused on basic issues of social justice, you may be met with a wide range of topics that may be irrelevant to your research. However, if you approach this data set with the specific intent of investigating opinions on gender issues, you will be able to focus on this topic alone, which would allow you to code only what you need to investigate.
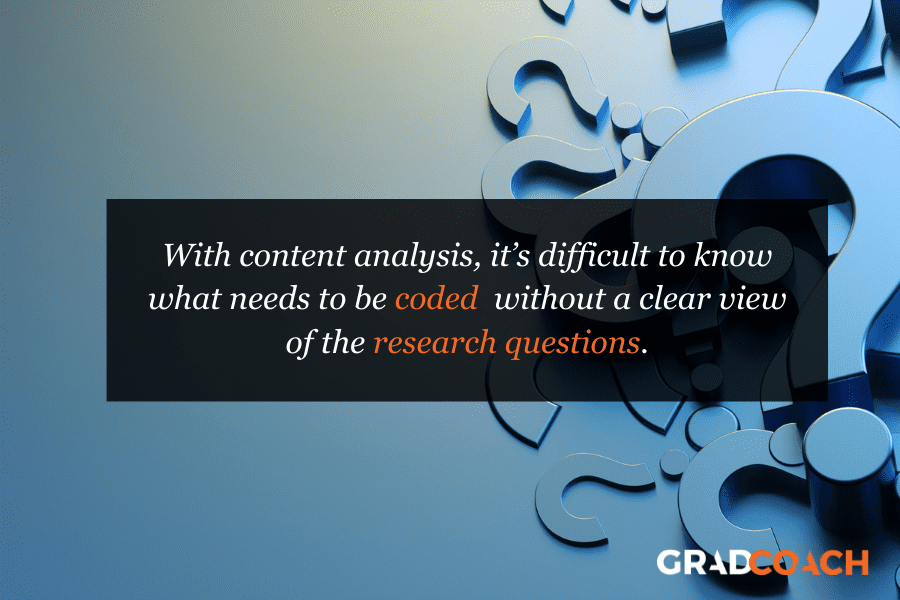
Step 2 – Reflect on your personal perspectives and biases
It’s vital that you reflect on your own pre-conception of the topic at hand and identify the biases that you might drag into your content analysis – this is called “ bracketing “. By identifying this upfront, you’ll be more aware of them and less likely to have them subconsciously influence your analysis.
For example, if you were to investigate how a community converses about unequal access to healthcare, it is important to assess your views to ensure that you don’t project these onto your understanding of the opinions put forth by the community. If you have access to medical aid, for instance, you should not allow this to interfere with your examination of unequal access.
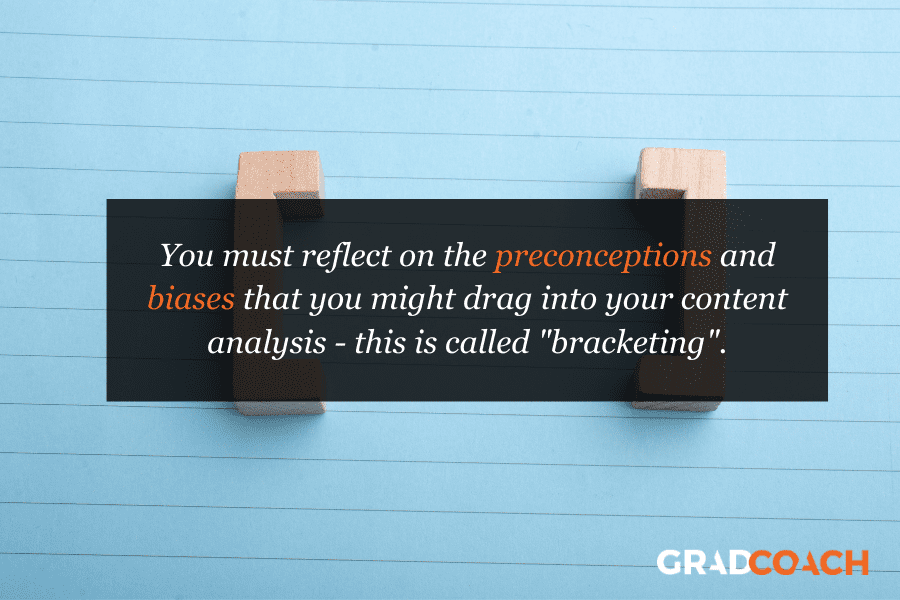
Step 3 – Operationalise your variables and develop a coding scheme
Next, you need to operationalise your variables . But what does that mean? Simply put, it means that you have to define each variable or construct . Give every item a clear definition – what does it mean (include) and what does it not mean (exclude). For example, if you were to investigate children’s views on healthy foods, you would first need to define what age group/range you’re looking at, and then also define what you mean by “healthy foods”.
In combination with the above, it is important to create a coding scheme , which will consist of information about your variables (how you defined each variable), as well as a process for analysing the data. For this, you would refer back to how you operationalised/defined your variables so that you know how to code your data.
For example, when coding, when should you code a food as “healthy”? What makes a food choice healthy? Is it the absence of sugar or saturated fat? Is it the presence of fibre and protein? It’s very important to have clearly defined variables to achieve consistent coding – without this, your analysis will get very muddy, very quickly.
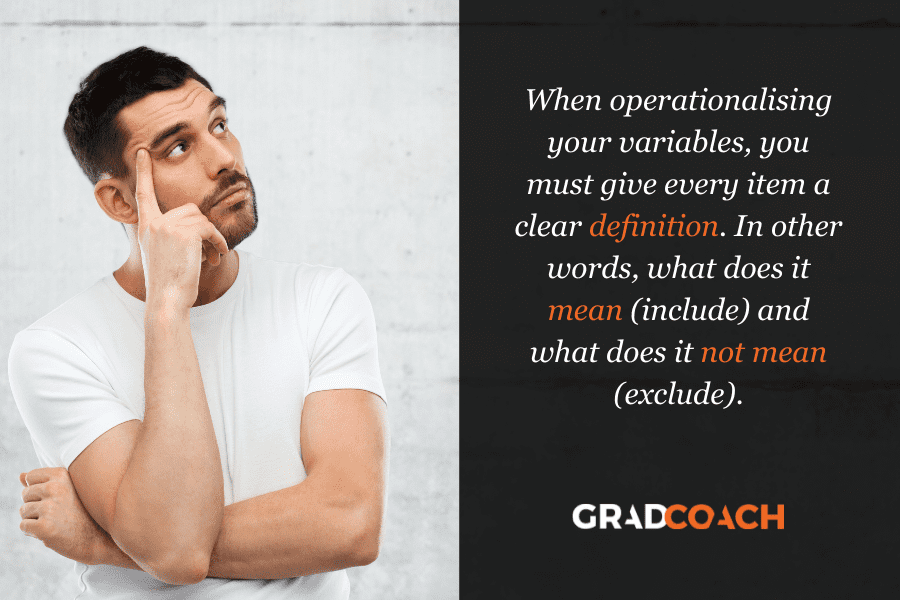
Step 4 – Code and analyse the data
The next step is to code the data. At this stage, there are some differences between conceptual and relational analysis.
As described earlier in this post, conceptual analysis looks at the existence and frequency of concepts, whereas a relational analysis looks at the relationships between concepts. For both types of analyses, it is important to pre-select a concept that you wish to assess in your data. Using the example of studying children’s views on healthy food, you could pre-select the concept of “healthy food” and assess the number of times the concept pops up in your data.
Here is where conceptual and relational analysis start to differ.
At this stage of conceptual analysis , it is necessary to decide on the level of analysis you’ll perform on your data, and whether this will exist on the word, phrase, sentence, or thematic level. For example, will you code the phrase “healthy food” on its own? Will you code each term relating to healthy food (e.g., broccoli, peaches, bananas, etc.) with the code “healthy food” or will these be coded individually? It is very important to establish this from the get-go to avoid inconsistencies that could result in you having to code your data all over again.
On the other hand, relational analysis looks at the type of analysis. So, will you use affect extraction? Proximity analysis? Cognitive mapping? A mix? It’s vital to determine the type of analysis before you begin to code your data so that you can maintain the reliability and validity of your research .
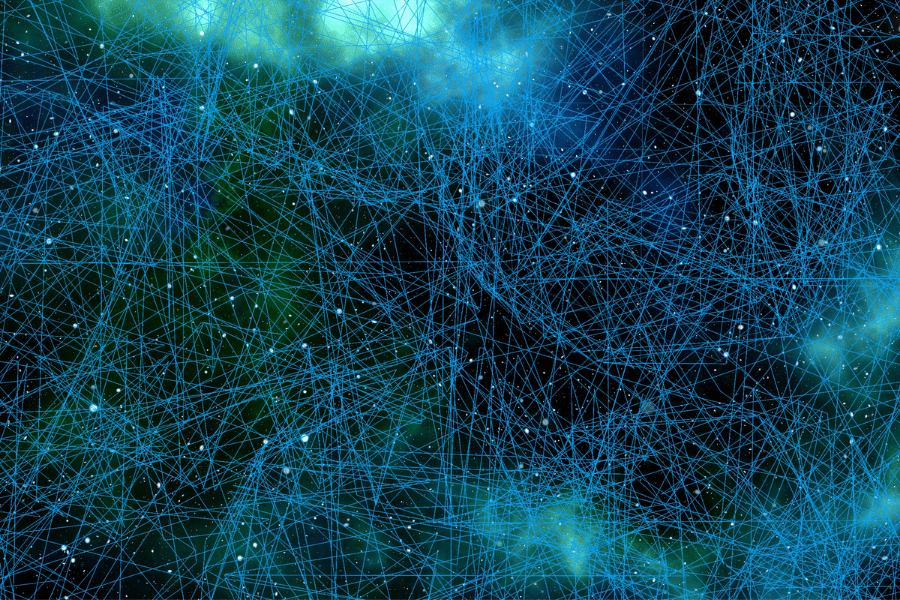
How to conduct conceptual analysis
First, let’s have a look at the process for conceptual analysis.
Once you’ve decided on your level of analysis, you need to establish how you will code your concepts, and how many of these you want to code. Here you can choose whether you want to code in a deductive or inductive manner. Just to recap, deductive coding is when you begin the coding process with a set of pre-determined codes, whereas inductive coding entails the codes emerging as you progress with the coding process. Here it is also important to decide what should be included and excluded from your analysis, and also what levels of implication you wish to include in your codes.
For example, if you have the concept of “tall”, can you include “up in the clouds”, derived from the sentence, “the giraffe’s head is up in the clouds” in the code, or should it be a separate code? In addition to this, you need to know what levels of words may be included in your codes or not. For example, if you say, “the panda is cute” and “look at the panda’s cuteness”, can “cute” and “cuteness” be included under the same code?
Once you’ve considered the above, it’s time to code the text . We’ve already published a detailed post about coding , so we won’t go into that process here. Once you’re done coding, you can move on to analysing your results. This is where you will aim to find generalisations in your data, and thus draw your conclusions .
How to conduct relational analysis
Now let’s return to relational analysis.
As mentioned, you want to look at the relationships between concepts . To do this, you’ll need to create categories by reducing your data (in other words, grouping similar concepts together) and then also code for words and/or patterns. These are both done with the aim of discovering whether these words exist, and if they do, what they mean.
Your next step is to assess your data and to code the relationships between your terms and meanings, so that you can move on to your final step, which is to sum up and analyse the data.
To recap, it’s important to start your analysis process by reviewing your research questions and identifying your biases . From there, you need to operationalise your variables, code your data and then analyse it.
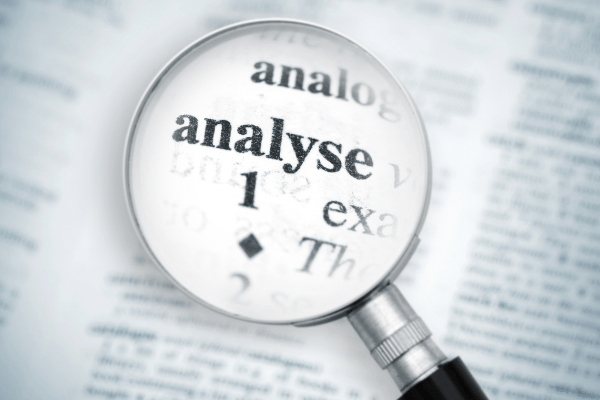
5. What are the pros & cons of content analysis?
One of the main advantages of content analysis is that it allows you to use a mix of quantitative and qualitative research methods, which results in a more scientifically rigorous analysis.
For example, with conceptual analysis, you can count the number of times that a term or a code appears in a dataset, which can be assessed from a quantitative standpoint. In addition to this, you can then use a qualitative approach to investigate the underlying meanings of these and relationships between them.
Content analysis is also unobtrusive and therefore poses fewer ethical issues than some other analysis methods. As the content you’ll analyse oftentimes already exists, you’ll analyse what has been produced previously, and so you won’t have to collect data directly from participants. When coded correctly, data is analysed in a very systematic and transparent manner, which means that issues of replicability (how possible it is to recreate research under the same conditions) are reduced greatly.
On the downside , qualitative research (in general, not just content analysis) is often critiqued for being too subjective and for not being scientifically rigorous enough. This is where reliability (how replicable a study is by other researchers) and validity (how suitable the research design is for the topic being investigated) come into play – if you take these into account, you’ll be on your way to achieving sound research results.
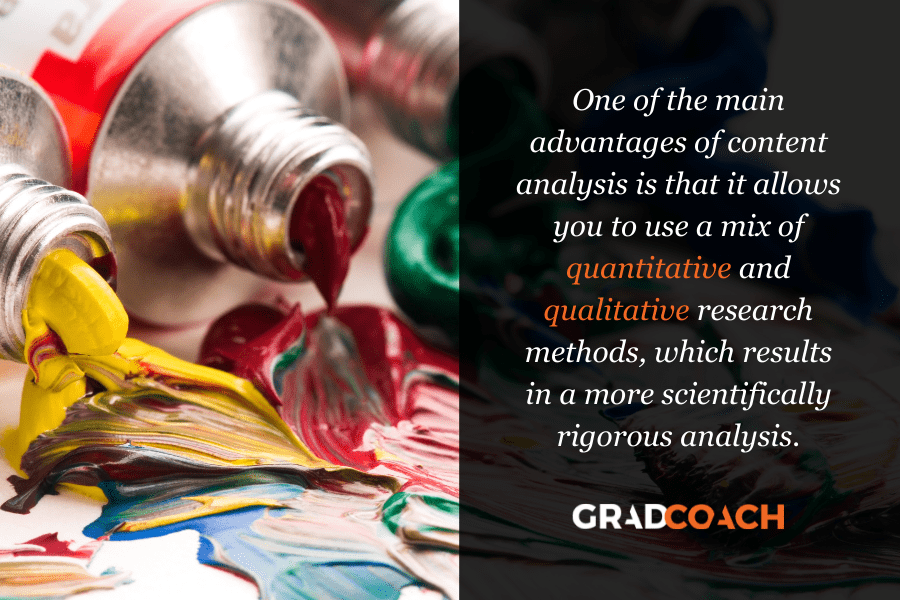
Recap: Qualitative content analysis
In this post, we’ve covered a lot of ground – click on any of the sections to recap:
If you have any questions about qualitative content analysis, feel free to leave a comment below. If you’d like 1-on-1 help with your qualitative content analysis, be sure to book an initial consultation with one of our friendly Research Coaches.
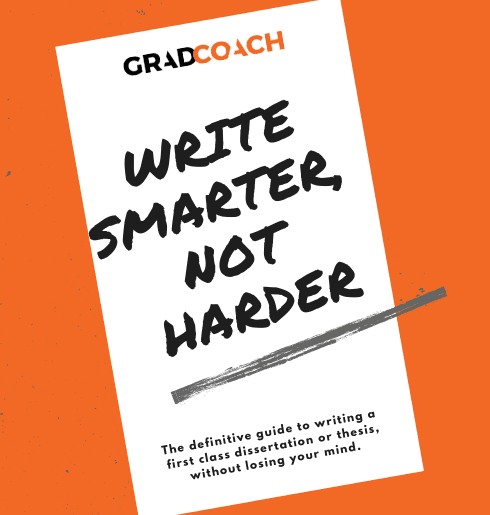
Psst… there’s more (for free)
This post is part of our dissertation mini-course, which covers everything you need to get started with your dissertation, thesis or research project.
You Might Also Like:
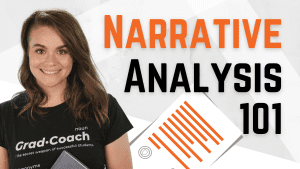
13 Comments
If I am having three pre-decided attributes for my research based on which a set of semi-structured questions where asked then should I conduct a conceptual content analysis or relational content analysis. please note that all three attributes are different like Agility, Resilience and AI.
Thank you very much. I really enjoyed every word.
please send me one/ two sample of content analysis
send me to any sample of qualitative content analysis as soon as possible
Many thanks for the brilliant explanation. Do you have a sample practical study of a foreign policy using content analysis?
1) It will be very much useful if a small but complete content analysis can be sent, from research question to coding and analysis. 2) Is there any software by which qualitative content analysis can be done?
Common software for qualitative analysis is nVivo, and quantitative analysis is IBM SPSS
Thank you. Can I have at least 2 copies of a sample analysis study as my reference?
Could you please send me some sample of textbook content analysis?
Can I send you my research topic, aims, objectives and questions to give me feedback on them?
please could you send me samples of content analysis?
really we enjoyed your knowledge thanks allot. from Ethiopia
can you please share some samples of content analysis(relational)? I am a bit confused about processing the analysis part
Submit a Comment Cancel reply
Your email address will not be published. Required fields are marked *
Save my name, email, and website in this browser for the next time I comment.
- Print Friendly

10 Content Analysis Examples
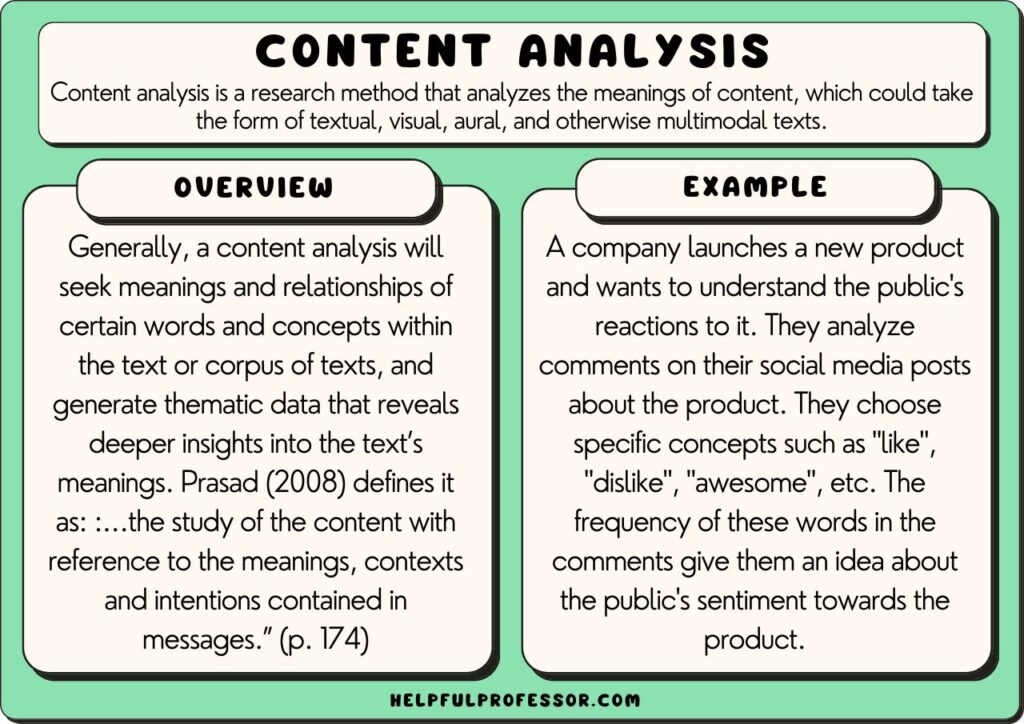
Content analysis is a research method and type of textual analysis that analyzes the meanings of content , which could take the form of textual, visual, aural, and otherwise multimodal texts.
Generally, a content analysis will seek meanings and relationships of certain words and concepts within the text or corpus of texts, and generate thematic data that reveals deeper insights into the text’s meanings.
Prasad (2008) defines it as:
:…the study of the content with reference to the meanings, contexts and intentions contained in messages.” (p. 174)
Content analyses can involve deductive coding , where themes and concepts are asserted before the content is created; or, they can involve inductive coding , where themes and concepts emerge during a close reading of the text.
An example of a content analysis would be a study that analyzes the presence of ideological words and phrases in newspapers to ascertain the editorial team’s political biases.
Content Analysis Examples
1. conceptual analysis.
Also called semantic content analysis, a conceptual analysis selects a concept and tries to count its occurrence within a text (Kosterec, 2016).
An example of a concept that you might examine is sentiment, such as positive, negative, and neutral sentiment. Here, you would need to conduct a semantic study of the text to find instances of words like ‘bad’, ‘terrible’, etc. for negative sentiment, and ‘good’, ‘great’, etc. for positive sentiment. A compare and contrast will demonstrate a balance of sentiment within the text.
A basic conceptual analysis has the weakness of lacking the capacity to read words in context, which would require a deeper qualitative analysis of paragraphs, which is offset by other types of analysis in this list.
Example of Conceptual Analysis
A company launches a new product and wants to understand the public’s initial reactions to it. They use conceptual analysis to analyze comments on their social media posts about the product. They could choose specific concepts such as “like”, “dislike”, “awesome”, “terrible”, etc. The frequency of these words in the comments give them an idea about the public’s sentiment towards the product.
2. Relational Analysis
Relational analysis addresses the above-mentioned weakness of conceptual analysis (i.e. that a mere counting of instances of terms lacks context) by examining how concepts in a text relate to one another .
Here, a scholar might analyze the overlap or sequences between certain concepts and sentiments in language (Kosterec, 2016). To combine the two examples from the above conceptual analysis, a scholar might examine all of a particular masthead newspaper’s columns on global warming. In the study, they would examine the proximity between the word ‘global warming’ and positive, negative, and neutral sentiment words (‘good’, ‘bad’, ‘great’, etc.) to ascertain the newspaper’s sentiment toward a specific concept .
Example of Relational Analysis
A political scientist wants to understand the relationship between the use of emotional rhetoric and audience reaction in political speeches. They carry out a relational analysis on a corpus of speeches and corresponding audience feedback. By exploring the co-occurrence of emotive words (“hope”, “fear”, “pride”) and audience responses (“applause”, “boos”, “silence”), they discover patterns in how different types of emotional language affect audience reactions.
3. Thematic Analysis
A thematic analysis focuses on identifying themes or major ideas running throughout the text.
This can follow a range of strategies, spanning from highly quantitative – such as using statistical software to thematically group words and terms – through to highly qualitative, where trained researchers take notes on each paragraph to extract key ideas that can be thematicized.
Many literature reviews take the form of a thematic analysis, where the scholar reads all recent studies on a topic and tries to ascertain themes, as well as gaps, across the recent literature.
Example of Thematic Analysis
A scholar searches on research bases for all published academic papers containing the keyword “back pain” from the past 10 years. She then uses inductive coding to generate themes that span the studies. From this thematic analysis, she produces a literature review on key emergent themes from the literature on back pain, as well as gaps in the research.
4. Narrative Analysis
This involves a close reading of the framing and structure of narrative elements within content. It can examine personal life stories, biographies, journals, and so on.
In literary research, this method generally explores the elements of the story , such as characters, plot, literary themes , and settings. But in life history research, it will generally involve deconstructing a real person’s life story, analyzing their perspectives and worldview to develop insights into their unique situation, life circumstances, or personality.
The focus generally expands out from the story itself to what it can tell us about the individuals or culture from which it originates.
Example of Narrative Analysis
A social work researcher takes a group of their patients’ personal journals and, after obtaining ethics clearance and permission from the patients, deconstructs the underlying messages in their journals in order to extract an understanding of the core mental hurdles each patient faces, which are then analyzed through the lens of Jungian psychoanalysis.
5. Discourse Analysis
Discourse analysis, the research methodology from which I conducted my PhD studies, involves the study of how language can create and reproduce social realities.
Based on the work of postmodern scholars such as Michel Foucault and Jaques Derrida, it attempts to deconstruct how texts normalize ways of thinking within specific historical, cultural, and social contexts .
Foucault, the most influential scholar in discourse analytic research, demonstrated through the study of how society spoke about madness that different societies constructed madness in different ways: in the renaissance era, mad people we spoken of as wise people, during the classical era, language changed, and they were framed as pariahs. Finally, in the modern era, they were spoken about as if they were sick.
Following Foucault (1988), many content analysis scholars now look at the differing ways societies frame different identities (gender, race, social class, etc.) in different times – and this can be revealed by looking at the language used in the content (i.e. the texts) produced throughout different eras (Johnstone, 2017).
Example of Discourse Analysis
A scholar examines a corpus of immigration speeches from a specific political party from the past 10 years and examines how refugees are discussed in the speeches, with a focus on how language constructs and defines refugees. It finds that refugees appear to be constructed as threats, dirty, and nefarious.
See Here for 10 More Examples of Discourse Analysis
6. Multimodal Analysis
As audiovisual texts became more important in society, many scholars began to critique the fact that content analysis tends to only look at written texts. In response, a methodology called multimodal analysis emerged.
In multimodal analysis, scholars don’t just decode the meanings in written texts, but also in multimodal texts . This involves the study of the signs, symbols, movements, and sounds that are within the text.
This opens up space for the analysis of television advertisements, billboards, and so forth.
For an example, a multimodal analysis of a television advertisement might not just study what is said, but it’ll explore how the camera angles frame some people as powerful (low to high angle) and some people as weak (high to low angle). Similarly, they may examine the colors to see if a character is positioned as sad (dark colors, walking through rain) or joyful (bright colors, sunshine).
Example of Multimodal Analysis
A cultural studies scholar examines the representation of Gender in Disney films, looking not only at the spoken words, but also the dresses worn, the camera angles, and the princesses’ tone of voice when speaking to other characters to assess how Disney’s construction of gender has changed over time.
7. Semiotic Analysis
Semiotic analysis takes multimodal analysis to the next step by providing the specific methods for the analysis of multimodal texts.
Seminal scholars Kress and van Leeuwen (2006) have created a significant repertoire of texts demonstrating how semiotics shape meaning. In their works, they present deconstructions of various modes of address:
- Visual: How images, signs, and symbols create meaning in social contexts. For example, in our modern world, a red octagon has a specific social meaning: stop!
- Textual: How words shape meaning, such as through a sentiment analysis as discussed earlier.
- Motive: How movement can create a sense of pace, distance, the movement of time, and so forth, which shapes meaning.
- Aural: How sounds shape meaning. For example, the words spoken are not the only way we interpret a speech, but also how they’re spoken (shakily, confidently, assertively, etc.)
Example of Semiotic Analysis
A communications studies scholar examines the body language of leaders during meetings at an international political event, using it to explore how the leaders subtly send messages about who they are allied with, where they view themselves in geopolitical terms, and their attitudes toward the event overall.
8. Latent Content Analysis
This involves the interpretation of the underlying, inferred meanings of the words or visuals. The focus here is on what is being implied by the content rather than just what is explicitly said.
For example, in the context of the same newspaper articles, a latent content analysis might examine the way the event is framed, the language or rhetoric used, the themes or narratives that are implied, or the attitudes and ideologies that are expressed or endorsed, either overtly or covertly .
Returning to the work of Foucault, he demonstrated how silence also constructs meaning. The question emerges: what is left unsaid in the content, and how does this shape our understanding of the biases and assumptions of the author?
Example of Latent Content Analysis
A sociologist studying gender roles in films watches the top 10 movies from last year and doesn’t just count instances of words – rather, they analyze the underlying, implicit messages about gender roles. This could include exploring how female characters are portrayed (do they tend to be passive and in need of rescue, or are they active, independent and resourceful?) and how male characters are portrayed (emotional or unemotional?) What kind of occupations do characters of each gender typically have?
9. Manifest Content Analysis
A manifest content analysis is the counterpoint to latent content analysis. It involves a direct and surface-level reading of the visible aspects of the content.
It concerns itself primarily with what is visible, obvious and countable. This approach asserts that we should not read too deeply into anything beyond what is manifest (i.e. present), because the deeper we try to read into the missing or latent elements, the more we stray into the real of guessing and assuming.
Scholars will often do both latent and manifest content analyses side-by-side, exploring how each type of analysis might reveal different interpretations or insights.
Example of Manifest Content Analysis
A researcher is interested in studying bias in media coverage of a particular political event. They might conduct a conceptual analysis where the concept is the tone of language used – positive, neutral, or negative. They would examine a number of articles from different newspapers, tallying up instances of positive, negative, or neutral language to see if there is a bias towards positivity or negativity in coverage of the event.
10. Longitudinal Content Analysis
A longitudinal content analysis analyzes trends in content over a long period of time.
Earlier, I explored the idea in discourse analysis that different eras have different ideas about terms and concepts (consider, for example, evolving ideas of gender and race). A longitudinal analysis would be very useful here. It would involve collecting cross-sectional moments in time , at varying points in time, which would then be compared and contrasted for the representation of varying concepts and terms.
Example of Longitudinal Content Analsis
A scholar might look at newspaper reports on texts from each decade for 100 years, examining environmental terms (‘global warming’, ‘climate change’, ‘recycling’) to identify when and how environmental concepts entered public discourse.
For other Examples of Analysis, See Here
Content analysis is a form of empirical research that uses texts rather than interviews or naturalistic observation to gather data that can then be analyzed. There are a range of methods and approaches to the analysis of content, but their unifying feature is that they involve close readings of texts to identify concepts and themes that might be revealing of core or underlying messages within the content.
The above examples are not mutually exclusive types, but rather different approaches that researchers can use based on their specific goals and the nature of the data they are working with.
Foucault, M. (1988). Madness and civilization: A history of insanity in the age of reason . London: Vintage.
Johnstone, B. (2017). Discourse analysis . London: John Wiley & Sons.
Kosterec, M. (2016). Methods of conceptual analysis. Filozofia , 71 (3).
Kress, G., & Van Leeuwen, T. (2006). The grammar of visual design . London and New York: Routledge.
Prasad, B. D. (2008). Content analysis: A method of Social Science Research . In D.K. Lal Das (ed) Research Methods for Social Work, (pp.174-193). New Delhi: Rawat Publications.
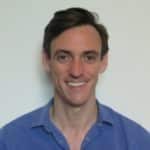
Chris Drew (PhD)
Dr. Chris Drew is the founder of the Helpful Professor. He holds a PhD in education and has published over 20 articles in scholarly journals. He is the former editor of the Journal of Learning Development in Higher Education. [Image Descriptor: Photo of Chris]
- Chris Drew (PhD) https://helpfulprofessor.com/author/chris-drew-phd/ 5 Top Tips for Succeeding at University
- Chris Drew (PhD) https://helpfulprofessor.com/author/chris-drew-phd/ 50 Durable Goods Examples
- Chris Drew (PhD) https://helpfulprofessor.com/author/chris-drew-phd/ 100 Consumer Goods Examples
- Chris Drew (PhD) https://helpfulprofessor.com/author/chris-drew-phd/ 30 Globalization Pros and Cons
Leave a Comment Cancel Reply
Your email address will not be published. Required fields are marked *
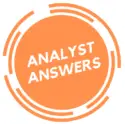
Data & Finance for Work & Life

Qualitative Content Analysis: a Simple Guide with Examples
Content analysis is a type of qualitative research (as opposed to quantitative research) that focuses on analyzing content in various mediums, the most common of which is written words in documents.
It’s a very common technique used in academia, especially for students working on theses and dissertations, but here we’re going to talk about how companies can use qualitative content analysis to improve their processes and increase revenue.
Whether you’re new to content analysis or a seasoned professor, this article provides all you need to know about how data analysts use content analysis to improve their business. It will also help you understand the relationship between content analysis and natural language processing — what some even call natural language content analysis.
Don’t forget, you can get the free Intro to Data Analysis eBook , which will ensure you build the right practical skills for success in your analytical endeavors.
What is qualitative content analysis, and what is it used for?
Any content analysis definition must consist of at least these three things: qualitative language , themes , and quantification .
In short, content analysis is the process of examining preselected words in video, audio, or written mediums and their context to identify themes, then quantifying them for statistical analysis in order to draw conclusions. More simply, it’s counting how often you see two words close to each other.
For example, let’s say I place in front of you an audio bit, a old video with a static image, and a document with lots of text but no titles or descriptions. At the start, you would have no idea what any of it was about.
Let’s say you transpose the video and audio recordings on paper. Then you use a counting software to count the top ten most used words, excluding prepositions (of, over, to, by) and articles (the, a), conjunctions (and, but, or) and other common words like “very.”
Your results are that the top 5 words are “candy,” “snow,” “cold,” and “sled.” These 5 words appear at least 25 times each, and the next highest word appears only 4 times. You also find that the words “snow” and “sled” appear adjacent to each other 95% of the time that “snow” appears.
Well, now you have performed a very elementary qualitative content analysis .
This means that you’re probably dealing with a text in which snow sleds are important. Snow sleds, thus, become a theme in these documents, which goes to the heart of qualitative content analysis.
The goal of qualitative content analysis is to organize text into a series of themes . This is opposed to quantitative content analysis, which aims to organize the text into categories .
Types of qualitative content analysis
If you’ve heard about content analysis, it was most likely in an academic setting. The term itself is common among PhD students and Masters students writing their dissertations and theses. In that context, the most common type of content analysis is document analysis.
There are many types of content analysis , including:
- Short- and long-form survey questions
- Focus group transcripts
- Interview transcripts
- Legislature
- Public records
- Comments sections
- Messaging platforms
This list gives you an idea for the possibilities and industries in which qualitative content analysis can be applied.
For example, marketing departments or public relations groups in major corporations might collect survey, focus groups, and interviews, then hand off the information to a data analyst who performs the content analysis.
A political analysis institution or Think Tank might look at legislature over time to identify potential emerging themes based on their slow introduction into policy margins. Perhaps it’s possible to identify certain beliefs in the senate and house of representatives before they enter the public discourse.
Non-governmental organizations (NGOs) might perform an analysis on public records to see how to better serve their constituents. If they have access to public records, it would be possible to identify citizen characteristics that align with their goal.
Analysis logic: inductive vs deductive
There are two types of logic we can apply to qualitative content analysis: inductive and deductive. Inductive content analysis is more of an exploratory approach. We don’t know what patterns or ideas we’ll discover, so we go in with an open mind.
On the other hand, deductive content analysis involves starting with an idea and identifying how it appears in the text. For example, we may approach legislation on wildlife by looking for rules on hunting. Perhaps we think hunting with a knife is too dangerous, and we want to identify trends in the text.
Neither one is better per se, and they each have carry value in different contexts. For example, inductive content analysis is advantageous in situations where we want to identify author intent. Going in with a hypothesis can bias the way we look at the data, so the inductive method is better
Deductive content analysis is better when we want to target a term. For example, if we want to see how important knife hunting is in the legislation, we’re doing deductive content analysis.
Measurements: idea coding vs word frequency
Two main methodologies exist for analyzing the text itself: coding and word frequency. Idea coding is the manual process of reading through a text and “coding” ideas in a column on the right. The reason we call this coding is because we take ideas and themes expressed in many words, and turn them into one common phrase. This allows researchers to better understand how those ideas evolve. We will look at how to do this in word below.
In short, coding in the context qualitative content analysis follows 2 steps:
- Reading through the text one time
- Adding 2-5 word summaries each time a significant theme or idea appears
Word frequency is simply counting the number of times a word appears in a text, as well as its proximity to other words. In our “snow sled” example above, we counted the number of times a word appeared, as well as how often it appeared next to other words. There’s are online tool for this we’ll look at below.
In short, word frequency in the context of content analysis follows 2 steps:
- Decide whether you want to find a word, or just look at the most common words
- Use word’s Replace function for the first, or an online tool such as Text Analyzer for the second (we’ll look at these in more detail below).
Many data scientists consider coding as the only qualitative content analysis, since word frequency turns to counting the number of times a word appears, making is quantitative.
While there is merit to this claim, I personally do not consider word frequency a part of quantitative content analysis. The fact that we count the frequency of a word does not mean we can draw direct conclusions from it. In fact, without a researcher to provide context on the number of time a word appears, word frequency is useless. True quantitative research carries conclusive value on its own.
Measurements AND analysis logic
There are four ways to approach qualitative content analysis given our two measurement types and inductive/deductive logical approaches. You could do inductive coding, inductive word frequency, deductive coding, and deductive word frequency.
The two best are inductive coding and deductive word frequency. If you would like to discover a document, trying to search for specific words will not inform you about its contents, so inductive word frequency is un-insightful.
Likewise, if you’re looking for the presence of a specific idea, you do not want to go through the whole document to code just to find it, so deductive coding is not insightful. Here’s simple matrix to illustrate:
Qualitative content analysis example
We looked at a small example above, but let’s play out all of the above information in a real world example. I will post the link to the text source at the bottom of the article, but don’t look at it yet . Let’s jump in with a discovery mentality , meaning let’s use an inductive approach and code our way through each paragraph.
Qualitative Content Analysis Example Download
*Click the “1” superscript to the right for a link to the source text. 1
How to do qualitative content analysis
We could use word frequency analysis to find out which are the most common x% of words in the text (deductive word frequency), but this takes some time because we need to build a formula that excludes words that are common but that don’t have any value (a, the, but, and, etc).
As a shortcut, you can use online tools such as Text Analyzer and WordCounter , which will give you breakdowns by phrase length (6 words, 5 words, 4 words, etc), without excluding common terms. Here are a few insightful example using our text with 7 words:

Perhaps more insightfully, here is a list of 5 word combinations, which are much more common:

The downside to these tools is that you cannot find 2- and 1-word strings without excluding common words. This is a limitation, but it’s unlikely that the work required to get there is worth the value it brings.
OK. Now that we’ve seen how to go about coding our text into quantifiable data, let’s look at the deductive approach and try to figure out if the text contains a single word we’re looking for. (This is my favorite.)
Deductive word frequency
We know the text now because we’ve already looked through it. It’s about the process of becoming literate, namely, the elements that impact our ability to learn to read. But we only looked at the first four sections of the article, so there’s more to explore.
Let’s say we want to know how a household situation might impact a student’s ability to read . Instead of coding the entire article, we can simply look for this term and it’s synonyms. The process for deductive word frequency is the following:
- Identify your term
- Think of all the possible synonyms
- Use the word find function to see how many times they appear
- If you suspect that this word often comes in connection with others, try searching for both of them
In my example, the process would be:
- Parents, parent, home, house, household situation, household influence, parental, parental situation, at home, home situation
- Go to “Edit>Find>Replace…” This will enable you to locate the number of instances in which your word or combinations appear. We use the Replace window instead of the simply Find bar because it allows us to visualize the information.
- Accounted for in possible synonyms
The results: 0! None of these words appeared in the text, so we can conclude that this text has nothing to do with a child’s home life and its impact on his/her ability to learn to read. Here’s a picture:

Don’t Be Afraid of Content Analysis
Content analysis can be intimidating because it uses data analysis to quantify words. This article provides a starting point for your analysis, but to ensure you get 90% reliability in word coding, sign up to receive our eBook Beginner Content Analysis . I went from philosophy student to a data-heavy finance career, and I created it to cater to research and dissertation use cases.

Content analysis vs natural language processing
While similar, content analysis, even the deductive word frequency approach, and natural language processing (NLP) are not the same. The relationship is hierarchical. Natural language processing is a field of linguistics and data science that’s concerned with understanding the meaning behind language.
On the other hand, content analysis is a branch of natural language processing that focuses on the methodologies we discussed above: discovery-style coding (sometimes called “tokenization”) and word frequency (sometimes called the “bag of words” technique)
For example, we would use natural language processing to quantify huge amounts of linguistic information, turn it into row-and-column data, and run tests on it. NLP is incredibly complex in the details, which is why it’s nearly impossible to provide a synopsis or example technique here (we’ll provide them in coursework on AnalystAnswers.com ). However, content analysis only focuses on a few manual techniques.
Content analysis in marketing
Content analysis in marketing is the use of content analysis to improve marketing reach and conversions. has grown in importance over the past ten years. As digital platforms become more central to our understanding and interaction with others, we use them more.
We write out ideas, small texts. We post our thoughts on Facebook and Twitter, and we write blog posts like this one. But we also post videos on youtube and express ourselves in podcasts.
All of these mediums contain valuable information about who we are and what we might want to buy . A good marketer aims to leverage this information in three ways:
- Collect the data
- Analyze the data
- Modify his/her marketing messaging to better serve the consumer
- Pretend, with bots or employees, to be a consumer and craft messages that influence potential buyers
The challenge for marketers doing this is getting the rights to access this data. Indeed, data privacy laws have gone into play in the European Union (General Data Protection Regulation, or GDPR) as well as in Brazil (General Data Protection Law, or GDPL).
Content analysis vs narrative analysis
Content analysis is concerned with themes and ideas, whereas narrative analysis is concerned with the stories people express about themselves or others. Narrative analysis uses the same tools as content analysis, namely coding (or tokenization) and word frequency, but its focus is on narrative relationship rather than themes. This is easier to understand with an example. Let’s look at how we might code the following paragraph from the two perspectives:
I do not like green eggs and ham. I do not like them, Sam-I-Am. I do not like them here or there. I do not like them anywhere!
Content analysis : the ideas expressed include green eggs and ham. the narrator does not like them
Narrative analysis : the narrator speaks from first person. He has a relationship with Sam-I-Am. He orients himself with regards to time and space. he does not like green eggs and ham, and may be willing to act on that feeling.
Content analysis vs document analysis
Content analysis and document analysis are very similar, which explains why many people use them interchangeably. The core difference is that content analysis examines all mediums in which words appear , whereas document analysis only examines written documents .
For example, if I want to carry out content analysis on a master’s thesis in education, I would consult documents, videos, and audio files. I may transcribe the video and audio files into a document, but I wouldn’t exclude them form the beginning.
On the other hand, if I want to carry out document analysis on a master’s thesis, I would only use documents, excluding the other mediums from the start. The methodology is the same, but the scope is different. This dichotomy also explains why most academic researchers performing qualitative content analysis refer to the process as “document analysis.” They rarely look at other mediums.
Content Gap Analysis
Content gap analysis is a term common in the field of content marketing, but it applies to the analytical fields as well. In a sentence, content gap analysis is the process of examining a document or text and identifying the missing pieces, or “gap,” that it needs to be completed.
As you can imagine, a content marketer uses gap analysis to determine how to improve blog content. An analyst uses it for other reasons. For example, he/she may have a standard for documents that merit analysis. If a document does not meet the criteria, it must be rejected until it’s improved.
The key message here is that content gap analysis is not content analysis. It’s a way of measuring the distance an underperforming document is from an acceptable document. It is sometimes, but not always, used in a qualitative content analysis context.
- Link to Source Text [ ↩ ]
About the Author
Noah is the founder & Editor-in-Chief at AnalystAnswers. He is a transatlantic professional and entrepreneur with 5+ years of corporate finance and data analytics experience, as well as 3+ years in consumer financial products and business software. He started AnalystAnswers to provide aspiring professionals with accessible explanations of otherwise dense finance and data concepts. Noah believes everyone can benefit from an analytical mindset in growing digital world. When he's not busy at work, Noah likes to explore new European cities, exercise, and spend time with friends and family.
File available immediately.
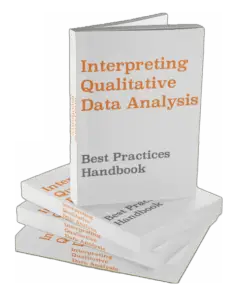
Notice: JavaScript is required for this content.
- Memberships
Content Analysis explained plus example

Content Analysis: this article provides a practical explanation of the term content analysis in the context of research skills. The article begins with a general definition and explanation, followed by a step-by-step plan for performing this type of analysis, including an example at each step. You will also find an overview of the benefits and drawbacks of applying such an analysis in a study. Enjoy reading!
What is a content analysis? A brief explanation
Content analysis is a research method that can identify patterns in recorded communications.
By systematically collecting data from different types of texts, such as written documents, speeches, web content or visual media, researchers can then discover valuable information about the goals , messages and effects of communication.

In this type of analysis, words, themes and concepts in the texts are categorized or “coded” , allowing for both quantitative and qualitative analyses.
Quantitative analysis focuses on counting and precisely measuring events, while qualitative analysis aims to interpret and understand the meaning and semantic relationships of words and concepts.
A Content Analysis example
An example. In a political campaign, content analysis can help researchers understand the importance of employment issues.
By analyzing political leaders’ campaign speeches, they can quantify the frequency of terms such as unemployment, jobs and employment and analyze them statistically for differences over time or between candidates.
In addition, they can also take a qualitative approach, examining the word unemployment in speeches, identifying related words or phrases and analyzing the meanings of these relationships. The aim is then to gain a deeper understanding of the intentions and target groups of different campaigns.
In which fields are content analyzes used?
Content analytics is applied in a variety of fields , including marketing , media studies, anthropology, cognitive science, psychology, and social sciences.
The goals of this type of analysis range from identifying correlations and patterns in communication to understanding intentions and analyzing the consequences of communication.
Research Methods For Business Students Course A-Z guide to writing a rockstar Research Paper with a bulletproof Research Methodology! More information
Step-by-step plan for performing a content analysis
Below you will find the steps that are followed when performing a content analysis.
Please note that the approach to the analysis may differ per situation. The steps you read about below are general steps.
Step 1: Select the content you want to analyze
Start by choosing specific texts or material to examine in the content analysis. This may include sources such as news articles, social media posts, interviews or documents. When analyzing social media posts, make sure you use social media analytics tools for gathering insights and measuring the performance of your content.
When analyzing social media posts, make sure you use social media analytics tools for gathering insights and measuring the performance of your content.
Example: Select a collection of 100 newspaper articles from reputable sources that deal with climate change.
Step 2: Define the units and categories of analysis
Determine the specific units within the content you want to analyze and create categories to classify them. Units can be individual words, sentences, paragraphs, or even entire sections.
Example: Define a unit as a single sentence in the newspaper articles and create categories such as “causes of climate change” , “impacts on the environment” and “policy recommendations” .
Step 3: Develop a set of coding rules
Create a clear and consistent set of guidelines for assigning codes to the units based on the established categories. These rules ensure consistent and reliable coding during analysis.
Example: Code a sentence discussing the role of greenhouse gasses as “GG” and a sentence pointing to renewable energy solutions as “RES” .
Step 4: Code the text according to the rules
Systematically apply the coding rules to the content and assign the correct codes to each unit.
Please read and interpret the content carefully to determine the most accurate code based on established guidelines.
Example: Read each sentence in the newspaper articles and assign the relevant code (GG or RES) to indicate whether the sentence discusses greenhouse gases or renewable energy solutions.
Step 5: Analyze the results from the Content Analysis and draw conclusions
Once coding is complete, analyze the encoded data to identify patterns, frequencies, and relationships between categories.
Calculate percentages or frequencies to quantify the occurrences of specific codes. Interpret the results to draw meaningful conclusions about the content.
Example: Calculate the percentage of sentences related to “causes of climate change” versus “policy recommendations” to understand the focus of the articles.
Analyze how often the code GG occurs compared to the code RES to assess the emphasis on renewable energy solutions.
Sampling as a part of the content analysis
Sampling is an important aspect of content analysis. Sampling determines which texts are included in the analysis.
There are several sampling methods that researchers can use:
Random sampling
In this method, texts are randomly selected from the population. Random sampling ensures that the selected texts are representative of the larger population.
It reduces the risk of bias and allows generalization of the findings to a broader context.
Example: In a content analysis of customer reviews on an e-commerce platform, random sampling can be used to obtain a random selection of reviews from the full pool of available reviews.
Purposive sampling
In purposive sampling, texts are selected based on specific criteria or characteristics relevant to the research purposes.
Researchers deliberately select texts that offer valuable insights or illustrate certain themes or perspectives.
Example: In an analysis of political speeches, purposive sampling can be used to select speeches by major political figures or to choose speeches that address specific policy issues relevant to the study.
Sampling units
Sampling units refer to the elements within the selected texts that will be analyzed. Researchers must determine the appropriate size and scope of analysis by defining the sampling units.
These units can vary depending on the research question, such as individual words, sentences, paragraphs or entire documents.
Example: In a content analysis of online news articles, the sampling units might be individual paragraphs within the articles. Researchers may choose to analyze the statements in these paragraphs.
Benefits of content analysis in research
The benefits are:
Objectivity
Content analysis uses a systematic and structured approach, making it possible to obtain objective and reproducible results . It provides a quantitative basis for analysis and interpretation.
Large amount of data
Content analysis enables researchers to efficiently analyze large amounts of texts. This allows them to spot patterns and trends that would otherwise be difficult to identify.
Flexibility
Content analysis can be applied to different types of texts, such as written, oral or visual sources. This makes it a versatile method that can be adapted to various research questions and disciplines.
Wide applicability
Content analysis is used in various fields such as marketing, media research, sociology, psychology and more. It offers a valuable tool for gaining insight into communication processes and their effects.
Drawbacks of content analysis in research
The use of content analysis also has some drawbacks. Read them below.
Limited to selected content
These types of analysis relies on the availability of recorded communications, such as written documents, interviews, social media posts, etc. It may limit interpretation to what is actually captured and may miss important contextual information.
Subjectivity of the Content Analysis
Because content analysis requires the interpretation of the researcher in coding and analyzing the data, there can be a degree of subjectivity. The researcher’s personal biases or interpretations may affect the results.
Time intensive
These types of analysis can be a time-consuming method, especially when analyzing large amounts of texts. It requires patience and accuracy in coding and analyzing the data.
Possible coding issues

Now it’s your turn
What do you think? Do you recognize the explanation about content analysis? Have you ever used content analysis for research? What challenges did you face while performing this analysis? How important do you think it is to have a representative sample when conducting a content analysis? What other things do you think researchers can use to ensure the reliability of the content analysis? Do you have tips or comments?
Share your experience and knowledge in the comments box below.
More information
- Barick, R. (2021). Research Methods For Business Students . Retrieved 02/16/2024 from Udemy.
- Drisko, J. W., & Maschi, T. (2016). Content analysis . Pocket Guide to Social Work .
- Krippendorff, K. (2018). Content analysis: An introduction to its methodology . Sage publications .
- Stemler, S. (2000). An overview of content analysis . Practical assessment, research, and evaluation, 7(1), 17.
- Weber, R. P. (1990). Basic content analysis (Vol. 49). Sage.
How to cite this article: Janse, B. (2023). Content Analysis . Retrieved [insert date] from Toolshero: https://www.toolshero.com/research/content-analysis/
Original publication date: 11/14/2023 | Last update: 02/09/2024
Add a link to this page on your website: <a href=” https://www.toolshero.com/research/content-analysis/”> Toolshero: Content Analysis</a>
Did you find this article interesting?
Your rating is more than welcome or share this article via Social media!
Average rating 4 / 5. Vote count: 4
No votes so far! Be the first to rate this post.
We are sorry that this post was not useful for you!
Let us improve this post!
Tell us how we can improve this post?
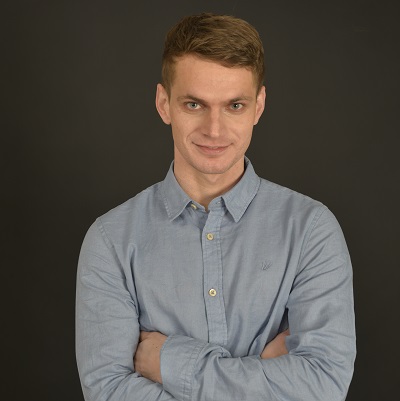
Ben Janse is a young professional working at ToolsHero as Content Manager. He is also an International Business student at Rotterdam Business School where he focusses on analyzing and developing management models. Thanks to his theoretical and practical knowledge, he knows how to distinguish main- and side issues and to make the essence of each article clearly visible.
Related ARTICLES
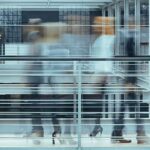
Gartner Magic Quadrant report and basics explained
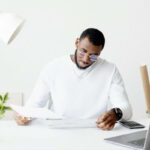
Univariate Analysis: basic theory and example
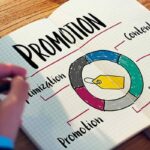
Content Marketing explained including strategy


Bivariate Analysis in Research explained
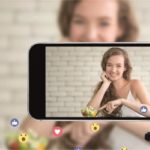
Influencer Marketing: Basics and Strategy explained
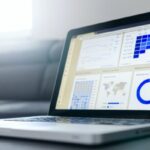
Contingency Table: the Theory and an Example
Also interesting.

Field Research explained
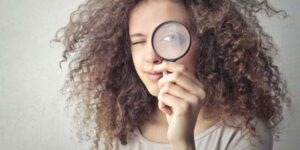
Observational Research Method explained
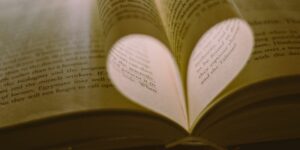
Research Ethics explained
Leave a reply cancel reply.
You must be logged in to post a comment.
BOOST YOUR SKILLS
Toolshero supports people worldwide ( 10+ million visitors from 100+ countries ) to empower themselves through an easily accessible and high-quality learning platform for personal and professional development.
By making access to scientific knowledge simple and affordable, self-development becomes attainable for everyone, including you! Join our learning platform and boost your skills with Toolshero.

POPULAR TOPICS
- Change Management
- Marketing Theories
- Problem Solving Theories
- Psychology Theories
ABOUT TOOLSHERO
- Free Toolshero e-book
- Memberships & Pricing
- Privacy Policy
Buy Me a Coffee

Home » Research Paper – Structure, Examples and Writing Guide
Research Paper – Structure, Examples and Writing Guide
Table of Contents

Research Paper
Definition:
Research Paper is a written document that presents the author’s original research, analysis, and interpretation of a specific topic or issue.
It is typically based on Empirical Evidence, and may involve qualitative or quantitative research methods, or a combination of both. The purpose of a research paper is to contribute new knowledge or insights to a particular field of study, and to demonstrate the author’s understanding of the existing literature and theories related to the topic.
Structure of Research Paper
The structure of a research paper typically follows a standard format, consisting of several sections that convey specific information about the research study. The following is a detailed explanation of the structure of a research paper:
The title page contains the title of the paper, the name(s) of the author(s), and the affiliation(s) of the author(s). It also includes the date of submission and possibly, the name of the journal or conference where the paper is to be published.
The abstract is a brief summary of the research paper, typically ranging from 100 to 250 words. It should include the research question, the methods used, the key findings, and the implications of the results. The abstract should be written in a concise and clear manner to allow readers to quickly grasp the essence of the research.
Introduction
The introduction section of a research paper provides background information about the research problem, the research question, and the research objectives. It also outlines the significance of the research, the research gap that it aims to fill, and the approach taken to address the research question. Finally, the introduction section ends with a clear statement of the research hypothesis or research question.
Literature Review
The literature review section of a research paper provides an overview of the existing literature on the topic of study. It includes a critical analysis and synthesis of the literature, highlighting the key concepts, themes, and debates. The literature review should also demonstrate the research gap and how the current study seeks to address it.
The methods section of a research paper describes the research design, the sample selection, the data collection and analysis procedures, and the statistical methods used to analyze the data. This section should provide sufficient detail for other researchers to replicate the study.
The results section presents the findings of the research, using tables, graphs, and figures to illustrate the data. The findings should be presented in a clear and concise manner, with reference to the research question and hypothesis.
The discussion section of a research paper interprets the findings and discusses their implications for the research question, the literature review, and the field of study. It should also address the limitations of the study and suggest future research directions.
The conclusion section summarizes the main findings of the study, restates the research question and hypothesis, and provides a final reflection on the significance of the research.
The references section provides a list of all the sources cited in the paper, following a specific citation style such as APA, MLA or Chicago.
How to Write Research Paper
You can write Research Paper by the following guide:
- Choose a Topic: The first step is to select a topic that interests you and is relevant to your field of study. Brainstorm ideas and narrow down to a research question that is specific and researchable.
- Conduct a Literature Review: The literature review helps you identify the gap in the existing research and provides a basis for your research question. It also helps you to develop a theoretical framework and research hypothesis.
- Develop a Thesis Statement : The thesis statement is the main argument of your research paper. It should be clear, concise and specific to your research question.
- Plan your Research: Develop a research plan that outlines the methods, data sources, and data analysis procedures. This will help you to collect and analyze data effectively.
- Collect and Analyze Data: Collect data using various methods such as surveys, interviews, observations, or experiments. Analyze data using statistical tools or other qualitative methods.
- Organize your Paper : Organize your paper into sections such as Introduction, Literature Review, Methods, Results, Discussion, and Conclusion. Ensure that each section is coherent and follows a logical flow.
- Write your Paper : Start by writing the introduction, followed by the literature review, methods, results, discussion, and conclusion. Ensure that your writing is clear, concise, and follows the required formatting and citation styles.
- Edit and Proofread your Paper: Review your paper for grammar and spelling errors, and ensure that it is well-structured and easy to read. Ask someone else to review your paper to get feedback and suggestions for improvement.
- Cite your Sources: Ensure that you properly cite all sources used in your research paper. This is essential for giving credit to the original authors and avoiding plagiarism.
Research Paper Example
Note : The below example research paper is for illustrative purposes only and is not an actual research paper. Actual research papers may have different structures, contents, and formats depending on the field of study, research question, data collection and analysis methods, and other factors. Students should always consult with their professors or supervisors for specific guidelines and expectations for their research papers.
Research Paper Example sample for Students:
Title: The Impact of Social Media on Mental Health among Young Adults
Abstract: This study aims to investigate the impact of social media use on the mental health of young adults. A literature review was conducted to examine the existing research on the topic. A survey was then administered to 200 university students to collect data on their social media use, mental health status, and perceived impact of social media on their mental health. The results showed that social media use is positively associated with depression, anxiety, and stress. The study also found that social comparison, cyberbullying, and FOMO (Fear of Missing Out) are significant predictors of mental health problems among young adults.
Introduction: Social media has become an integral part of modern life, particularly among young adults. While social media has many benefits, including increased communication and social connectivity, it has also been associated with negative outcomes, such as addiction, cyberbullying, and mental health problems. This study aims to investigate the impact of social media use on the mental health of young adults.
Literature Review: The literature review highlights the existing research on the impact of social media use on mental health. The review shows that social media use is associated with depression, anxiety, stress, and other mental health problems. The review also identifies the factors that contribute to the negative impact of social media, including social comparison, cyberbullying, and FOMO.
Methods : A survey was administered to 200 university students to collect data on their social media use, mental health status, and perceived impact of social media on their mental health. The survey included questions on social media use, mental health status (measured using the DASS-21), and perceived impact of social media on their mental health. Data were analyzed using descriptive statistics and regression analysis.
Results : The results showed that social media use is positively associated with depression, anxiety, and stress. The study also found that social comparison, cyberbullying, and FOMO are significant predictors of mental health problems among young adults.
Discussion : The study’s findings suggest that social media use has a negative impact on the mental health of young adults. The study highlights the need for interventions that address the factors contributing to the negative impact of social media, such as social comparison, cyberbullying, and FOMO.
Conclusion : In conclusion, social media use has a significant impact on the mental health of young adults. The study’s findings underscore the need for interventions that promote healthy social media use and address the negative outcomes associated with social media use. Future research can explore the effectiveness of interventions aimed at reducing the negative impact of social media on mental health. Additionally, longitudinal studies can investigate the long-term effects of social media use on mental health.
Limitations : The study has some limitations, including the use of self-report measures and a cross-sectional design. The use of self-report measures may result in biased responses, and a cross-sectional design limits the ability to establish causality.
Implications: The study’s findings have implications for mental health professionals, educators, and policymakers. Mental health professionals can use the findings to develop interventions that address the negative impact of social media use on mental health. Educators can incorporate social media literacy into their curriculum to promote healthy social media use among young adults. Policymakers can use the findings to develop policies that protect young adults from the negative outcomes associated with social media use.
References :
- Twenge, J. M., & Campbell, W. K. (2019). Associations between screen time and lower psychological well-being among children and adolescents: Evidence from a population-based study. Preventive medicine reports, 15, 100918.
- Primack, B. A., Shensa, A., Escobar-Viera, C. G., Barrett, E. L., Sidani, J. E., Colditz, J. B., … & James, A. E. (2017). Use of multiple social media platforms and symptoms of depression and anxiety: A nationally-representative study among US young adults. Computers in Human Behavior, 69, 1-9.
- Van der Meer, T. G., & Verhoeven, J. W. (2017). Social media and its impact on academic performance of students. Journal of Information Technology Education: Research, 16, 383-398.
Appendix : The survey used in this study is provided below.
Social Media and Mental Health Survey
- How often do you use social media per day?
- Less than 30 minutes
- 30 minutes to 1 hour
- 1 to 2 hours
- 2 to 4 hours
- More than 4 hours
- Which social media platforms do you use?
- Others (Please specify)
- How often do you experience the following on social media?
- Social comparison (comparing yourself to others)
- Cyberbullying
- Fear of Missing Out (FOMO)
- Have you ever experienced any of the following mental health problems in the past month?
- Do you think social media use has a positive or negative impact on your mental health?
- Very positive
- Somewhat positive
- Somewhat negative
- Very negative
- In your opinion, which factors contribute to the negative impact of social media on mental health?
- Social comparison
- In your opinion, what interventions could be effective in reducing the negative impact of social media on mental health?
- Education on healthy social media use
- Counseling for mental health problems caused by social media
- Social media detox programs
- Regulation of social media use
Thank you for your participation!
Applications of Research Paper
Research papers have several applications in various fields, including:
- Advancing knowledge: Research papers contribute to the advancement of knowledge by generating new insights, theories, and findings that can inform future research and practice. They help to answer important questions, clarify existing knowledge, and identify areas that require further investigation.
- Informing policy: Research papers can inform policy decisions by providing evidence-based recommendations for policymakers. They can help to identify gaps in current policies, evaluate the effectiveness of interventions, and inform the development of new policies and regulations.
- Improving practice: Research papers can improve practice by providing evidence-based guidance for professionals in various fields, including medicine, education, business, and psychology. They can inform the development of best practices, guidelines, and standards of care that can improve outcomes for individuals and organizations.
- Educating students : Research papers are often used as teaching tools in universities and colleges to educate students about research methods, data analysis, and academic writing. They help students to develop critical thinking skills, research skills, and communication skills that are essential for success in many careers.
- Fostering collaboration: Research papers can foster collaboration among researchers, practitioners, and policymakers by providing a platform for sharing knowledge and ideas. They can facilitate interdisciplinary collaborations and partnerships that can lead to innovative solutions to complex problems.
When to Write Research Paper
Research papers are typically written when a person has completed a research project or when they have conducted a study and have obtained data or findings that they want to share with the academic or professional community. Research papers are usually written in academic settings, such as universities, but they can also be written in professional settings, such as research organizations, government agencies, or private companies.
Here are some common situations where a person might need to write a research paper:
- For academic purposes: Students in universities and colleges are often required to write research papers as part of their coursework, particularly in the social sciences, natural sciences, and humanities. Writing research papers helps students to develop research skills, critical thinking skills, and academic writing skills.
- For publication: Researchers often write research papers to publish their findings in academic journals or to present their work at academic conferences. Publishing research papers is an important way to disseminate research findings to the academic community and to establish oneself as an expert in a particular field.
- To inform policy or practice : Researchers may write research papers to inform policy decisions or to improve practice in various fields. Research findings can be used to inform the development of policies, guidelines, and best practices that can improve outcomes for individuals and organizations.
- To share new insights or ideas: Researchers may write research papers to share new insights or ideas with the academic or professional community. They may present new theories, propose new research methods, or challenge existing paradigms in their field.
Purpose of Research Paper
The purpose of a research paper is to present the results of a study or investigation in a clear, concise, and structured manner. Research papers are written to communicate new knowledge, ideas, or findings to a specific audience, such as researchers, scholars, practitioners, or policymakers. The primary purposes of a research paper are:
- To contribute to the body of knowledge : Research papers aim to add new knowledge or insights to a particular field or discipline. They do this by reporting the results of empirical studies, reviewing and synthesizing existing literature, proposing new theories, or providing new perspectives on a topic.
- To inform or persuade: Research papers are written to inform or persuade the reader about a particular issue, topic, or phenomenon. They present evidence and arguments to support their claims and seek to persuade the reader of the validity of their findings or recommendations.
- To advance the field: Research papers seek to advance the field or discipline by identifying gaps in knowledge, proposing new research questions or approaches, or challenging existing assumptions or paradigms. They aim to contribute to ongoing debates and discussions within a field and to stimulate further research and inquiry.
- To demonstrate research skills: Research papers demonstrate the author’s research skills, including their ability to design and conduct a study, collect and analyze data, and interpret and communicate findings. They also demonstrate the author’s ability to critically evaluate existing literature, synthesize information from multiple sources, and write in a clear and structured manner.
Characteristics of Research Paper
Research papers have several characteristics that distinguish them from other forms of academic or professional writing. Here are some common characteristics of research papers:
- Evidence-based: Research papers are based on empirical evidence, which is collected through rigorous research methods such as experiments, surveys, observations, or interviews. They rely on objective data and facts to support their claims and conclusions.
- Structured and organized: Research papers have a clear and logical structure, with sections such as introduction, literature review, methods, results, discussion, and conclusion. They are organized in a way that helps the reader to follow the argument and understand the findings.
- Formal and objective: Research papers are written in a formal and objective tone, with an emphasis on clarity, precision, and accuracy. They avoid subjective language or personal opinions and instead rely on objective data and analysis to support their arguments.
- Citations and references: Research papers include citations and references to acknowledge the sources of information and ideas used in the paper. They use a specific citation style, such as APA, MLA, or Chicago, to ensure consistency and accuracy.
- Peer-reviewed: Research papers are often peer-reviewed, which means they are evaluated by other experts in the field before they are published. Peer-review ensures that the research is of high quality, meets ethical standards, and contributes to the advancement of knowledge in the field.
- Objective and unbiased: Research papers strive to be objective and unbiased in their presentation of the findings. They avoid personal biases or preconceptions and instead rely on the data and analysis to draw conclusions.
Advantages of Research Paper
Research papers have many advantages, both for the individual researcher and for the broader academic and professional community. Here are some advantages of research papers:
- Contribution to knowledge: Research papers contribute to the body of knowledge in a particular field or discipline. They add new information, insights, and perspectives to existing literature and help advance the understanding of a particular phenomenon or issue.
- Opportunity for intellectual growth: Research papers provide an opportunity for intellectual growth for the researcher. They require critical thinking, problem-solving, and creativity, which can help develop the researcher’s skills and knowledge.
- Career advancement: Research papers can help advance the researcher’s career by demonstrating their expertise and contributions to the field. They can also lead to new research opportunities, collaborations, and funding.
- Academic recognition: Research papers can lead to academic recognition in the form of awards, grants, or invitations to speak at conferences or events. They can also contribute to the researcher’s reputation and standing in the field.
- Impact on policy and practice: Research papers can have a significant impact on policy and practice. They can inform policy decisions, guide practice, and lead to changes in laws, regulations, or procedures.
- Advancement of society: Research papers can contribute to the advancement of society by addressing important issues, identifying solutions to problems, and promoting social justice and equality.
Limitations of Research Paper
Research papers also have some limitations that should be considered when interpreting their findings or implications. Here are some common limitations of research papers:
- Limited generalizability: Research findings may not be generalizable to other populations, settings, or contexts. Studies often use specific samples or conditions that may not reflect the broader population or real-world situations.
- Potential for bias : Research papers may be biased due to factors such as sample selection, measurement errors, or researcher biases. It is important to evaluate the quality of the research design and methods used to ensure that the findings are valid and reliable.
- Ethical concerns: Research papers may raise ethical concerns, such as the use of vulnerable populations or invasive procedures. Researchers must adhere to ethical guidelines and obtain informed consent from participants to ensure that the research is conducted in a responsible and respectful manner.
- Limitations of methodology: Research papers may be limited by the methodology used to collect and analyze data. For example, certain research methods may not capture the complexity or nuance of a particular phenomenon, or may not be appropriate for certain research questions.
- Publication bias: Research papers may be subject to publication bias, where positive or significant findings are more likely to be published than negative or non-significant findings. This can skew the overall findings of a particular area of research.
- Time and resource constraints: Research papers may be limited by time and resource constraints, which can affect the quality and scope of the research. Researchers may not have access to certain data or resources, or may be unable to conduct long-term studies due to practical limitations.
About the author
Muhammad Hassan
Researcher, Academic Writer, Web developer
You may also like

How to Cite Research Paper – All Formats and...

Data Collection – Methods Types and Examples

Delimitations in Research – Types, Examples and...

Research Paper Format – Types, Examples and...

Research Process – Steps, Examples and Tips

Research Design – Types, Methods and Examples

An official website of the United States government
The .gov means it’s official. Federal government websites often end in .gov or .mil. Before sharing sensitive information, make sure you’re on a federal government site.
The site is secure. The https:// ensures that you are connecting to the official website and that any information you provide is encrypted and transmitted securely.
- Publications
- Account settings
Preview improvements coming to the PMC website in October 2024. Learn More or Try it out now .
- Advanced Search
- Journal List
- Afr J Emerg Med
- v.7(3); 2017 Sep
A hands-on guide to doing content analysis
Christen erlingsson.
a Department of Health and Caring Sciences, Linnaeus University, Kalmar 391 82, Sweden
Petra Brysiewicz
b School of Nursing & Public Health, University of KwaZulu-Natal, Durban 4041, South Africa
Associated Data
There is a growing recognition for the important role played by qualitative research and its usefulness in many fields, including the emergency care context in Africa. Novice qualitative researchers are often daunted by the prospect of qualitative data analysis and thus may experience much difficulty in the data analysis process. Our objective with this manuscript is to provide a practical hands-on example of qualitative content analysis to aid novice qualitative researchers in their task.
African relevance
- • Qualitative research is useful to deepen the understanding of the human experience.
- • Novice qualitative researchers may benefit from this hands-on guide to content analysis.
- • Practical tips and data analysis templates are provided to assist in the analysis process.
Introduction
There is a growing recognition for the important role played by qualitative research and its usefulness in many fields, including emergency care research. An increasing number of health researchers are currently opting to use various qualitative research approaches in exploring and describing complex phenomena, providing textual accounts of individuals’ “life worlds”, and giving voice to vulnerable populations our patients so often represent. Many articles and books are available that describe qualitative research methods and provide overviews of content analysis procedures [1] , [2] , [3] , [4] , [5] , [6] , [7] , [8] , [9] , [10] . Some articles include step-by-step directions intended to clarify content analysis methodology. What we have found in our teaching experience is that these directions are indeed very useful. However, qualitative researchers, especially novice researchers, often struggle to understand what is happening on and between steps, i.e., how the steps are taken.
As research supervisors of postgraduate health professionals, we often meet students who present brilliant ideas for qualitative studies that have potential to fill current gaps in the literature. Typically, the suggested studies aim to explore human experience. Research questions exploring human experience are expediently studied through analysing textual data e.g., collected in individual interviews, focus groups, documents, or documented participant observation. When reflecting on the proposed study aim together with the student, we often suggest content analysis methodology as the best fit for the study and the student, especially the novice researcher. The interview data are collected and the content analysis adventure begins. Students soon realise that data based on human experiences are complex, multifaceted and often carry meaning on multiple levels.
For many novice researchers, analysing qualitative data is found to be unexpectedly challenging and time-consuming. As they soon discover, there is no step-wise analysis process that can be applied to the data like a pattern cutter at a textile factory. They may become extremely annoyed and frustrated during the hands-on enterprise of qualitative content analysis.
The novice researcher may lament, “I’ve read all the methodology but don’t really know how to start and exactly what to do with my data!” They grapple with qualitative research terms and concepts, for example; differences between meaning units, codes, categories and themes, and regarding increasing levels of abstraction from raw data to categories or themes. The content analysis adventure may now seem to be a chaotic undertaking. But, life is messy, complex and utterly fascinating. Experiencing chaos during analysis is normal. Good advice for the qualitative researcher is to be open to the complexity in the data and utilise one’s flow of creativity.
Inspired primarily by descriptions of “conventional content analysis” in Hsieh and Shannon [3] , “inductive content analysis” in Elo and Kyngäs [5] and “qualitative content analysis of an interview text” in Graneheim and Lundman [1] , we have written this paper to help the novice qualitative researcher navigate the uncertainty in-between the steps of qualitative content analysis. We will provide advice and practical tips, as well as data analysis templates, to attempt to ease frustration and hopefully, inspire readers to discover how this exciting methodology contributes to developing a deeper understanding of human experience and our professional contexts.
Overview of qualitative content analysis
Synopsis of content analysis.
A common starting point for qualitative content analysis is often transcribed interview texts. The objective in qualitative content analysis is to systematically transform a large amount of text into a highly organised and concise summary of key results. Analysis of the raw data from verbatim transcribed interviews to form categories or themes is a process of further abstraction of data at each step of the analysis; from the manifest and literal content to latent meanings ( Fig. 1 and Table 1 ).

Example of analysis leading to higher levels of abstraction; from manifest to latent content.
Glossary of terms as used in this hands-on guide to doing content analysis. *
The initial step is to read and re-read the interviews to get a sense of the whole, i.e., to gain a general understanding of what your participants are talking about. At this point you may already start to get ideas of what the main points or ideas are that your participants are expressing. Then one needs to start dividing up the text into smaller parts, namely, into meaning units. One then condenses these meaning units further. While doing this, you need to ensure that the core meaning is still retained. The next step is to label condensed meaning units by formulating codes and then grouping these codes into categories. Depending on the study’s aim and quality of the collected data, one may choose categories as the highest level of abstraction for reporting results or you can go further and create themes [1] , [2] , [3] , [5] , [8] .
Content analysis as a reflective process
You must mould the clay of the data , tapping into your intuition while maintaining a reflective understanding of how your own previous knowledge is influencing your analysis, i.e., your pre-understanding. In qualitative methodology, it is imperative to vigilantly maintain an awareness of one’s pre-understanding so that this does not influence analysis and/or results. This is the difficult balancing task of keeping a firm grip on one’s assumptions, opinions, and personal beliefs, and not letting them unconsciously steer your analysis process while simultaneously, and knowingly, utilising one’s pre-understanding to facilitate a deeper understanding of the data.
Content analysis, as in all qualitative analysis, is a reflective process. There is no “step 1, 2, 3, done!” linear progression in the analysis. This means that identifying and condensing meaning units, coding, and categorising are not one-time events. It is a continuous process of coding and categorising then returning to the raw data to reflect on your initial analysis. Are you still satisfied with the length of meaning units? Do the condensed meaning units and codes still “fit” with each other? Do the codes still fit into this particular category? Typically, a fair amount of adjusting is needed after the first analysis endeavour. For example: a meaning unit might need to be split into two meaning units in order to capture an additional core meaning; a code modified to more closely match the core meaning of the condensed meaning unit; or a category name tweaked to most accurately describe the included codes. In other words, analysis is a flexible reflective process of working and re-working your data that reveals connections and relationships. Once condensed meaning units are coded it is easier to get a bigger picture and see patterns in your codes and organise codes in categories.
Content analysis exercise
The synopsis above is representative of analysis descriptions in many content analysis articles. Although correct, such method descriptions still do not provide much support for the novice researcher during the actual analysis process. Aspiring to provide guidance and direction to support the novice, a practical example of doing the actual work of content analysis is provided in the following sections. This practical example is based on a transcribed interview excerpt that was part of a study that aimed to explore patients’ experiences of being admitted into the emergency centre ( Fig. 2 ).

Excerpt from interview text exploring “Patient’s experience of being admitted into the emergency centre”
This content analysis exercise provides instructions, tips, and advice to support the content analysis novice in a) familiarising oneself with the data and the hermeneutic spiral, b) dividing up the text into meaning units and subsequently condensing these meaning units, c) formulating codes, and d) developing categories and themes.
Familiarising oneself with the data and the hermeneutic spiral
An important initial phase in the data analysis process is to read and re-read the transcribed interview while keeping your aim in focus. Write down your initial impressions. Embrace your intuition. What is the text talking about? What stands out? How did you react while reading the text? What message did the text leave you with? In this analysis phase, you are gaining a sense of the text as a whole.
You may ask why this is important. During analysis, you will be breaking down the whole text into smaller parts. Returning to your notes with your initial impressions will help you see if your “parts” analysis is matching up with your first impressions of the “whole” text. Are your initial impressions visible in your analysis of the parts? Perhaps you need to go back and check for different perspectives. This is what is referred to as the hermeneutic spiral or hermeneutic circle. It is the process of comparing the parts to the whole to determine whether impressions of the whole verify the analysis of the parts in all phases of analysis. Each part should reflect the whole and the whole should be reflected in each part. This concept will become clearer as you start working with your data.
Dividing up the text into meaning units and condensing meaning units
You have now read the interview a number of times. Keeping your research aim and question clearly in focus, divide up the text into meaning units. Located meaning units are then condensed further while keeping the central meaning intact ( Table 2 ). The condensation should be a shortened version of the same text that still conveys the essential message of the meaning unit. Sometimes the meaning unit is already so compact that no further condensation is required. Some content analysis sources warn researchers against short meaning units, claiming that this can lead to fragmentation [1] . However, our personal experience as research supervisors has shown us that a greater problem for the novice is basing analysis on meaning units that are too large and include many meanings which are then lost in the condensation process.
Suggestion for how the exemplar interview text can be divided into meaning units and condensed meaning units ( condensations are in parentheses ).
Formulating codes
The next step is to develop codes that are descriptive labels for the condensed meaning units ( Table 3 ). Codes concisely describe the condensed meaning unit and are tools to help researchers reflect on the data in new ways. Codes make it easier to identify connections between meaning units. At this stage of analysis you are still keeping very close to your data with very limited interpretation of content. You may adjust, re-do, re-think, and re-code until you get to the point where you are satisfied that your choices are reasonable. Just as in the initial phase of getting to know your data as a whole, it is also good to write notes during coding on your impressions and reactions to the text.
Suggestions for coding of condensed meaning units.
Developing categories and themes
The next step is to sort codes into categories that answer the questions who , what , when or where? One does this by comparing codes and appraising them to determine which codes seem to belong together, thereby forming a category. In other words, a category consists of codes that appear to deal with the same issue, i.e., manifest content visible in the data with limited interpretation on the part of the researcher. Category names are most often short and factual sounding.
In data that is rich with latent meaning, analysis can be carried on to create themes. In our practical example, we have continued the process of abstracting data to a higher level, from category to theme level, and developed three themes as well as an overarching theme ( Table 4 ). Themes express underlying meaning, i.e., latent content, and are formed by grouping two or more categories together. Themes are answering questions such as why , how , in what way or by what means? Therefore, theme names include verbs, adverbs and adjectives and are very descriptive or even poetic.
Suggestion for organisation of coded meaning units into categories and themes.
Some reflections and helpful tips
Understand your pre-understandings.
While conducting qualitative research, it is paramount that the researcher maintains a vigilance of non-bias during analysis. In other words, did you remain aware of your pre-understandings, i.e., your own personal assumptions, professional background, and previous experiences and knowledge? For example, did you zero in on particular aspects of the interview on account of your profession (as an emergency doctor, emergency nurse, pre-hospital professional, etc.)? Did you assume the patient’s gender? Did your assumptions affect your analysis? How about aspects of culpability; did you assume that this patient was at fault or that this patient was a victim in the crash? Did this affect how you analysed the text?
Staying aware of one’s pre-understandings is exactly as difficult as it sounds. But, it is possible and it is requisite. Focus on putting yourself and your pre-understandings in a holding pattern while you approach your data with an openness and expectation of finding new perspectives. That is the key: expect the new and be prepared to be surprised. If something in your data feels unusual, is different from what you know, atypical, or even odd – don’t by-pass it as “wrong”. Your reactions and intuitive responses are letting you know that here is something to pay extra attention to, besides the more comfortable condensing and coding of more easily recognisable meaning units.
Use your intuition
Intuition is a great asset in qualitative analysis and not to be dismissed as “unscientific”. Intuition results from tacit knowledge. Just as tacit knowledge is a hallmark of great clinicians [11] , [12] ; it is also an invaluable tool in analysis work [13] . Literally, take note of your gut reactions and intuitive guidance and remember to write these down! These notes often form a framework of possible avenues for further analysis and are especially helpful as you lift the analysis to higher levels of abstraction; from meaning units to condensed meaning units, to codes, to categories and then to the highest level of abstraction in content analysis, themes.
Aspects of coding and categorising hard to place data
All too often, the novice gets overwhelmed by interview material that deals with the general subject matter of the interview, but doesn’t seem to answer the research question. Don’t be too quick to consider such text as off topic or dross [6] . There is often data that, although not seeming to match the study aim precisely, is still important for illuminating the problem area. This can be seen in our practical example about exploring patients’ experiences of being admitted into the emergency centre. Initially the participant is describing the accident itself. While not directly answering the research question, the description is important for understanding the context of the experience of being admitted into the emergency centre. It is very common that participants will “begin at the beginning” and prologue their narratives in order to create a context that sets the scene. This type of contextual data is vital for gaining a deepened understanding of participants’ experiences.
In our practical example, the participant begins by describing the crash and the rescue, i.e., experiences leading up to and prior to admission to the emergency centre. That is why we have chosen in our analysis to code the condensed meaning unit “Ambulance staff looked worried about all the blood” as “In the ambulance” and place it in the category “Reliving the rescue”. We did not choose to include this meaning unit in the categories specifically about admission to the emergency centre itself. Do you agree with our coding choice? Would you have chosen differently?
Another common problem for the novice is deciding how to code condensed meaning units when the unit can be labelled in several different ways. At this point researchers usually groan and wish they had thought to ask one of those classic follow-up questions like “Can you tell me a little bit more about that?” We have examples of two such coding conundrums in the exemplar, as can be seen in Table 3 (codes we conferred on) and Table 4 (codes we reached consensus on). Do you agree with our choices or would you have chosen different codes? Our best advice is to go back to your impressions of the whole and lean into your intuition when choosing codes that are most reasonable and best fit your data.
A typical problem area during categorisation, especially for the novice researcher, is overlap between content in more than one initial category, i.e., codes included in one category also seem to be a fit for another category. Overlap between initial categories is very likely an indication that the jump from code to category was too big, a problem not uncommon when the data is voluminous and/or very complex. In such cases, it can be helpful to first sort codes into narrower categories, so-called subcategories. Subcategories can then be reviewed for possibilities of further aggregation into categories. In the case of a problematic coding, it is advantageous to return to the meaning unit and check if the meaning unit itself fits the category or if you need to reconsider your preliminary coding.
It is not uncommon to be faced by thorny problems such as these during coding and categorisation. Here we would like to reiterate how valuable it is to have fellow researchers with whom you can discuss and reflect together with, in order to reach consensus on the best way forward in your data analysis. It is really advantageous to compare your analysis with meaning units, condensations, coding and categorisations done by another researcher on the same text. Have you identified the same meaning units? Do you agree on coding? See similar patterns in the data? Concur on categories? Sometimes referred to as “researcher triangulation,” this is actually a key element in qualitative analysis and an important component when striving to ensure trustworthiness in your study [14] . Qualitative research is about seeking out variations and not controlling variables, as in quantitative research. Collaborating with others during analysis lets you tap into multiple perspectives and often makes it easier to see variations in the data, thereby enhancing the quality of your results as well as contributing to the rigor of your study. It is important to note that it is not necessary to force consensus in the findings but one can embrace these variations in interpretation and use that to capture the richness in the data.
Yet there are times when neither openness, pre-understanding, intuition, nor researcher triangulation does the job; for example, when analysing an interview and one is simply confused on how to code certain meaning units. At such times, there are a variety of options. A good starting place is to re-read all the interviews through the lens of this specific issue and actively search for other similar types of meaning units you might have missed. Another way to handle this is to conduct further interviews with specific queries that hopefully shed light on the issue. A third option is to have a follow-up interview with the same person and ask them to explain.
Additional tips
It is important to remember that in a typical project there are several interviews to analyse. Codes found in a single interview serve as a starting point as you then work through the remaining interviews coding all material. Form your categories and themes when all project interviews have been coded.
When submitting an article with your study results, it is a good idea to create a table or figure providing a few key examples of how you progressed from the raw data of meaning units, to condensed meaning units, coding, categorisation, and, if included, themes. Providing such a table or figure supports the rigor of your study [1] and is an element greatly appreciated by reviewers and research consumers.
During the analysis process, it can be advantageous to write down your research aim and questions on a sheet of paper that you keep nearby as you work. Frequently referring to your aim can help you keep focused and on track during analysis. Many find it helpful to colour code their transcriptions and write notes in the margins.
Having access to qualitative analysis software can be greatly helpful in organising and retrieving analysed data. Just remember, a computer does not analyse the data. As Jennings [15] has stated, “… it is ‘peopleware,’ not software, that analyses.” A major drawback is that qualitative analysis software can be prohibitively expensive. One way forward is to use table templates such as we have used in this article. (Three analysis templates, Templates A, B, and C, are provided as supplementary online material ). Additionally, the “find” function in word processing programmes such as Microsoft Word (Redmond, WA USA) facilitates locating key words, e.g., in transcribed interviews, meaning units, and codes.
Lessons learnt/key points
From our experience with content analysis we have learnt a number of important lessons that may be useful for the novice researcher. They are:
- • A method description is a guideline supporting analysis and trustworthiness. Don’t get caught up too rigidly following steps. Reflexivity and flexibility are just as important. Remember that a method description is a tool helping you in the process of making sense of your data by reducing a large amount of text to distil key results.
- • It is important to maintain a vigilant awareness of one’s own pre-understandings in order to avoid bias during analysis and in results.
- • Use and trust your own intuition during the analysis process.
- • If possible, discuss and reflect together with other researchers who have analysed the same data. Be open and receptive to new perspectives.
- • Understand that it is going to take time. Even if you are quite experienced, each set of data is different and all require time to analyse. Don’t expect to have all the data analysis done over a weekend. It may take weeks. You need time to think, reflect and then review your analysis.
- • Keep reminding yourself how excited you have felt about this area of research and how interesting it is. Embrace it with enthusiasm!
- • Let it be chaotic – have faith that some sense will start to surface. Don’t be afraid and think you will never get to the end – you will… eventually!
Peer review under responsibility of African Federation for Emergency Medicine.
Appendix A Supplementary data associated with this article can be found, in the online version, at http://dx.doi.org/10.1016/j.afjem.2017.08.001 .
Appendix A. Supplementary data
Content Analysis Research Paper
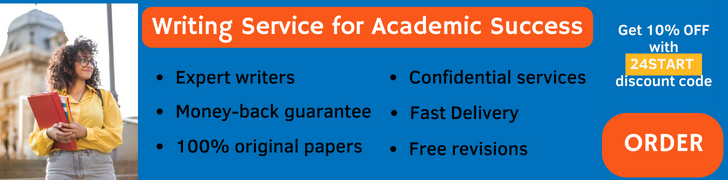
View sample Content Analysis Research Paper. Browse other research paper examples and check the list of political science research paper topics for more inspiration. If you need a research paper written according to all the academic standards, you can always turn to our experienced writers for help. This is how your paper can get an A! Also, chech our custom research proposal writing service for professional assistance. We offer high-quality assignments for reasonable rates.
I. Introduction
Academic writing, editing, proofreading, and problem solving services, get 10% off with 24start discount code, ii. what is content analysis, iii. forms of content analysis, iv. issues in designing content analysis studies, v. human coders, reliability, and stability, vi. computer-assisted content analysis, vii. future directions.
Content analysis is, as its name suggests, the analysis of the content of communications. Researchers use content analysis to make statements about the meaning, impact, or producers of those communications. Depending on the purpose of the specific research project, analysts may focus on the literal content or seek to extract deeper (or latent) meanings.
This multiplicity of purposes has led content analysts to use a variety of strategies for analyzing text systematically. Some of these strategies, such as word counts, are easy to replicate, whereas other forms are far more interpretive and dependent on the judgment of the individual who codes the text. Most forms of content analysis yield quantitative indicators. Indeed, some would define quantification as an essential aspect of content analysis (e.g., Weber, 1990). Others view it as preferable but not essential (Berelson, 1952; Holsti, 1969).
Content analysis is not new. According to Krippendorff (1980), empirical studies of communications can be dated back to the 1600s. More immediate ancestors to modern content analysis, however, are studies that sought to evaluate the content of mass media in the early 20th century and Nazi propaganda during World War II (Berelson, 1952; Krippendorff, 1980). As a method for studying communications, content analysis has been an especially popular methodology in the field of (mass) communication.
Holsti (1969) reported a trend toward a more frequent use of content analysis, as well as its application to a broader array of problems, including subjects of interest to political scientists. He furthermore noted an emerging tendency for content analysis to be used in combination with other social science research methods and a move toward computer-assisted content analysis.
This research paper emphasizes quantification, although it also discusses some of the trade-offs between quantitative and qualitative forms of content analysis. After discussing definitions and forms of content analysis, the paper describes some of the issues in designing content analysis studies, with the objective of giving the reader the basic tools for evaluating whether this methodology might be useful in her or his research. The paper then turns to the issue of reliability and stability, which are of particular importance when using human coders, and subsequently turns to a discussion of the emerging strategy of using computer assistance in the coding of text for content analysis. The paper ends with an assessment of the future of content analysis in political science.
According to Weber (1990), content analysis is a “research method that uses a set of procedures to make valid inferences from text” (p. 9). This concise definition captures the essence of content analysis very well, although it may be worth adding that text is not the only content that might be subjected to analysis. (Transcripts of) oral communications, as well as visual communications, could also be subjected to this type of analysis. This research paper, however, limits its scope to the content analysis of text (or at least verbal material) and does not consider the analysis of visual communications.
Beyond making valid inferences from text, most content analysis “seeks to quantify content in terms of predetermined categories and in a systematic and replicable manner” (Bryman, 2004, p. 181; Holsti, 1969). In other words, content analysis endeavors to analyze text in a systematic, empirical manner that is made sufficiently explicit to permit replication. Generally, this means that content analysis proceeds on the basis of instructions that enumerate explicit categories. Consequently, Babbie (2004) has described content analysis as “essentially a coding operation” (p. 318). Although this is accurate, it also sells content analysis short as a method for analyzing the content of communications.
The coding operation is at the heart of content analysis, but content analysis cannot be reduced to coding, just as public opinion research cannot be reduced to the survey instruments often used to ascertain public opinion. The coding of text or other communications permits the analyst to ascertain patterns and test hypotheses about those communications. Holsti (1969) maintained that content analysis “must be undertaken for some theoretical reason” (p. 14). Although not all studies employing content analysis satisfy that condition, such studies are generally undertaken to answer some question that is either of scientific interest or of political (or professional) relevance. In other words, studies may employ content analysis for a variety of purposes. Holsti summarized these purposes into three groupings:
- Content analysis may be used to describe characteristics of communications. For instance, a researcher may wish to discern trends in the content of newspaper or other media outlets or analyze the rhetorical style of a decision maker. An example of this type of research in political science is Breuning, Bredehoft, and Walton’s (2005) analysis of academic journal content.
- Content analysis may be used to infer psychological or other characteristics of the speaker. For instance, Hermann’s (1980, 2002) Leadership Trait Analysis is based on content analysis. She used a coding scheme that is informed by psychological theories to analyze the (spontaneous) remarks of political leaders in order to make assessments about various personality traits.
- It is further possible to use content analysis to assess the (potential) impact of communications. Eshbaugh Soha’s (2006) study of political impact of presidential speeches is an example of this type of analysis.
Within political science, content analysis has been used for all three of these purposes, although not with the same frequency. Studies that use content analysis to evaluate leader personality, motivation, or both, tend to be more plentiful in political science than studies of the impact of communication. Studies that describe the characteristics of communications tend to be more plentiful in the study of (mass) communication than in political science.
A distinct benefit of content analysis is that it is an unobtrusive research method (Babbie, 2004). The advantage of such a research method is that, one, it does not require the cooperation of the subject under investigation, and two, the subject will not alter her or his behavior as a result of awareness of being tested. The second point is important. There is evidence, for instance, that survey respondents on occasion provide socially acceptable answers rather than truthfully reporting their behavior. They may say they voted, because they think they should have, when in fact they stayed home. The first point is relevant to the study of political decision makers and foreign policy decision making. Although it can be useful to understand what motivates decision makers, it is highly unlikely that such individuals would make themselves available for psychological testing. Some researchers (e.g., Hermann, 1980, 2002; Walker, Schafer, & Young, 1998; Winter, 2005) have therefore devised research strategies that rely on content analysis of remarks and speeches in order to evaluate decision makers’ personalities and motivations. It is in this area of political science that content analysis is used most consistently.
Other benefits of content analysis include that it is relatively easy to undertake. It requires no special equipment or access to significant research funds (Babbie, 2004). Whereas survey research can be expensive, a study using content analysis can be completed for very little money. A single investigator with access to the relevant textual material for coding can complete a content analysis study, although a very large content analysis–based study may require multiple human coders or access to content analysis software in order to complete the study in a timely fashion. Paying human coders or purchasing content analysis software would, obviously, add to the expense of implementing a content analysis study.
Further, an investigator can much more easily repeat a portion of the study than would be the case with survey research (Babbie, 2004; Bryman, 2004). This includes the determination that an additional dimension needs to be considered in the analysis. It requires going back through the text to code the additional variable, which can be time-consuming when human coders are used, but it remains feasible as long as the text remains available. Repeating the analysis or adding another dimension to be coded is quite easy when a computer-assisted content analysis strategy is used.
Finally, content analysis lends itself to studying trends over long stretches of time (Bryman, 2004). For instance, Eshbaugh-Soha (2006) analyzed presidential speeches across a 50-year period.
All of these advantages make content analysis useful and attractive. On the other hand, the method is limited to the investigation of text and recorded human communications (Babbie, 2004; Bryman, 2004).
As already mentioned, content analysis generally refers to quantitative assessment of various aspects of text. This research paper places its emphasis on such analysis. As outlined previously, content analysis is defined by the quest to analyze text in a manner that is systematic, valid, and replicable. The first and last criteria are most easily satisfied through quantification. Although qualitative content analysis may be equally valid in its assessments of text, it is much less likely to be systematic, and it is exceedingly difficult to replicate. Although quantification can have important limitations, it has the distinct advantage of transparency: An explicitly formulated research design for a systematic and quantitative research design not only can be replicated but also allows any reader to evaluate how the investigator arrived at her or his conclusions.
Qualitative analysis does not inherently require such explicit research design and quite often depends on the expertise of the investigator (see, e.g., Neumann, 2008). The advantage of such analysis is that an investigator with substantive expertise may be able to identify nuances that a quantitative analysis misses. On the other hand, qualitative analysis, because it often lacks the sort of explicit coding scheme that is required for quantitative analysis, provides much less of a hedge against investigator bias. This led Babbie (2004) to suggest that an investigator engaged in qualitative content analysis must carefully search for disconfirming evidence—and report meticulously on any elements in the text that are inconsistent with the expected findings—to guard against a tendency to focus on the elements of the textual material that confirm the investigator’s expectations. If this is not done, there is the distinct risk that qualitative content analysis leads an investigator to confirm her or his expectations when these are not in fact supported by the evidence.
Quantitative content analysis, on the other hand, is more explicitly systematic and less dependent on the ability of an investigator to counteract the tendency to focus on confirming evidence. However, it may be less sensitive to contextual and cultural cues. In addition, the validity of quantitative content analysis depends on the adequacy of the coding scheme employed to analyze the text.
First, it is far easier to code manifest than latent content. Manifest content refers to the surface meaning of text, whereas latent content refers to the deeper or symbolic meaning. Content analysis has generally favored a focus on manifest content. Indeed, it has often been defined in terms of explicit and systematic coding rules (Holsti, 1969). In contrast, the analysis of the deeper, underlying meaning of text has more often been the province of those who favor qualitative content analysis or also discourse analysis (Neumann, 2008). As discussed previously, the latter type of analysis is less transparent, and replication is difficult or impossible. The focus on explicit and systematic coding rules does not mean that content analysis avoids interpretation but rather that it separates the data-gathering operation (the coding, counting, or both) from the interpretation of the results.
Second, the coding scheme needs to be carefully designed not only to ensure that it is explicitly stated and replicable, but also to ensure that it is grounded in the research question. In other words, the categories that are employed should be theoretically justified so that the resulting data help the investigator draw valid inferences from the text. What constitutes an appropriate coding scheme will depend on the research question the investigator seeks to test.
The distinction between manifest and latent content is perhaps somewhat artificial. Frequently, coding schemes that are based on relatively straightforward elements, such as word counts, do in fact seek to evaluate some aspect of latent content. In such cases, the specific words that are counted may have been chosen to reveal latent content.
For instance, in an analysis of the rhetoric of two ethnic nationalist Flemish parties in Belgium, Breuning and Ishiyama (1998) counted the references in the party platforms to the terms foreigners and immigrants. They theorized that the latter term has the connotation that the speaker or author perceives the persons who are being described as individuals who will integrate into the society and that the former describes individuals who are perceived as (permanent) outsiders. They further theorized that the extent to which a party preferred to use the term foreigner rather than immigrant when discussing nonnative populations was indicative the party’s xenophobia. In other words, Breuning and Ishiyama used word counts of specific terms to measure the differences in xenophobic attitude between these two ethnic nationalist parties. The coding scheme was transparent and easy to replicate but was designed to evaluate latent content.
The advantage of using carefully chosen but explicit coding schemes to reveal some aspect of latent content is that they are transparent and permit the researcher to show exactly how she or he arrived at the study’s interpretations and conclusions.
In addition to simple word counts, content analysis coding schemes may also record valences—that is, the value associated with the word that is coded. It does, after all, make a difference whether a word is used in a positive or negative construction. The automated coding scheme developed to study decision-makers’ operational codes includes valence measures (Walker et al., 1998).
Another variation is the use of thematic coding. In this case, the investigator is looking for evidence of specific themes in units of text. Theme coding can be tricky, since it involves judgment rather than simple counts. However, there are situations where thematic coding is theoretically justified—and word counts may simply not provide the investigator with an appropriate measure. In the creation of a coding scheme for thematic coding, extra care must be taken to make very clear and explicit the criteria for judging a unit of text to belong in one versus another category. Such a coding scheme may require pilot testing with several different coders to ensure that these coders share a common understanding of the coding instructions. The mechanics of designing a content analysis scheme are explored further in the next section of this research paper.
It can be difficult to devise a good content analysis study, although this also depends on the objective of the study. If the objective is to produce a study that is descriptive of some aspect of text, the construction of a coding scheme may not need to be overly complicated. In such a case, manifest content is coded to make systematic observations about that manifest content. At times, such studies have been accused of being atheoretical (Bryman, 2004). Whether this is a problem depends on the contribution a specific study seeks to make. For instance, Breuning et al. (2005) sought to analyze the content of academic journals and did not seek to test any theory. They simply sought to demonstrate in a quantifiable way who and what got published in the journals that they investigated. Such data provide insight into, for instance, the sort of work that is published in specific journals.
If, on the other hand, the study seeks to make inferences about a speaker or author or about the text’s impact, it can be much more difficult to determine what, exactly, needs to be coded. In that case, manifest content is coded in order to make inferences about underlying meaning or latent content. Content analysis studies that are undertaken for such objectives need to be thoroughly grounded in relevant theories, which can help to justify the validity of the measures. For instance, Hermann (1980, 2002) and Winter (2005) devised their content analysis schemes on the basis of psychological theories. Both sought to make inferences about the personality traits of decision makers on the basis of their interview responses and speeches.
How should an investigator go about designing a content analysis study, irrespective of whether she or he seeks to focus on manifest or latent content? There is not one single, correct way to design a content analysis study. The investigator needs to carefully consider a variety of issues in order to design a content analysis that helps her or him find answers to a specific research question. There are several sources that can provide guidance. Hermann (2002) presented a set of eight questions that are designed to help a researcher determine whether content analysis is an appropriate research strategy. Holsti (1969), Krippendorff (1980), and Weber (1990) each discussed many of the trade-offs and mechanical details of designing content analysis studies. All of these sources are useful for investigators who would like more detailed guidance in designing a content analysis study. What follows here is a condensed guide to the sort of issues that investigators need to consider in designing such studies. It is intended to enable the reader to evaluate whether this methodology might be appropriate in her or his own research projects.
The first step is to devise a research question and ask whether content analysis would be an appropriate research strategy. If the question can be answered using text or other communications, then a content analysis may well be a useful strategy. It is important to check at this early stage whether a sufficient volume of appropriate text is available and accessible and in what form it is accessible. If one plans to conduct a computer-assisted content analysis, it will be extremely useful if the materials are available in electronic format. Contemporary text is far more likely to be accessible in such a format than are older documents. This does not make it impossible to conduct a computer-assisted content analysis of older documents, but doing so would require converting such documents to a machine-readable electronic format. That is an extra step that might be time-consuming to complete.
The second step involves decisions about the type of analysis to conduct. Content analysis is about generating data to answer a specific research question. Given one’s research question, is a quantitative or a qualitative approach appropriate? Is it possible to count word frequencies? Or would a thematic coding scheme be more appropriate? It may initially seem easier to devise a thematic coding scheme, but remember that the data generated in this way are usually less easily replicated than word counts.
It is likely to be more difficult to construct vocabularies for a content analysis study that counts relevant words, because the specific words must be carefully chosen for what they can reveal about underlying meaning. Despite the greater effort that needs to go into designing such a coding scheme, it may be well worth the effort to do so. Word counts that are theoretically informed (and designed to capture the latent meaning of text) derive their validity from that theoretical basis. Furthermore, the explicit nature of word count coding schemes makes them easier to replicate as well as easier to complete through computer-assisted coding strategies.
A distinct advantage of using computer-assisted coding strategies is that reliability becomes a nonissue: The computer finds every instance of the words it is programmed to count, so there is no error. Human coders get fatigued, and their attention wanders, leading them to make mistakes.
Although theme coding can be an appropriate strategy, it is far more difficult to have a computer assist in the task. Theme coding therefore tends to involve human coders, who may perform the coding task in inconsistent ways. Just as in producing words counts, human coders who are looking for thematic content may get fatigued and make errors as a result. In addition, thematic coding involves a judgment of the presence or absence of a specific theme in a unit of text. Depending on how explicit the coding instructions are and how well trained the human coders are, such judgments may show variation across different human coders.
In both word count and thematic coding, therefore, it is useful to evaluate the quality of the coding operation by taking two measures: One measures the consistency of each human coder, and other measures the congruity of the decisions made by different human coders. The next section delves into these measures in more detail.
The third step involves creating the coding manual and coding schedule (Bryman, 2004). The former is a detailed and explicit set of instructions to coders. It explains what the unit of analysis is and includes all the possible categories for each dimension that will be coded. For word counts, it will list all the words that will be counted as being indicative of a specific dimension, including synonyms and variations of each word. For thematic coding, the coding manual should not only describe the categories, but also provide an example to help coders understand how to evaluate the text they will be coding.
It is extremely important that the categories for each dimension that will be coded be mutually exclusive and collectively exhaustive. This means that nothing should fit into more than one category simultaneously, and everything should fit into one of the categories. There should never be words, phrases, or themes that are part of a dimension that is being coded that cannot find a home in one of the categories. A clear and explicit coding manual is an important key to a successful content analysis study, especially if multiple coders work on the project, but it is also important to persuade others of the validity of the study.
The coding schedule is simply the form that the coder uses to record her or his coding decisions. Coding schedules may be paper, but it is also possible to use electronic spreadsheets or statistical analysis software instead. In the latter instance, the coder bypasses the need for data entry after completing the coding task.
A well-designed manual not only makes the coding task easier and the results more convincing but also ensures that the study is replicable. It is worthwhile to invest time and effort in the creation of a coding manual, pretesting it on a sample of text and revising it—multiple times if needed—to improve the coding categories or the clarity of the instructions, or both. In the process, it is useful to get feedback from others on the coding manual. If human coders will be completing much of the coding task, it also helps to have them each do a small pretest and check not only whether each coder implements the coding scheme as intended but also whether the different coders make substantially similar decisions.
As you ponder the various aspects of the design of a study, keep in mind whether the proposed analysis does indeed capture the goal of the study. Refer back to the research question and ask whether the coding manual will yield data that can reasonably be expected to shed light on that question.
A further issue to consider in designing the study is whether the text that will be analyzed is representational or instrumental (Hermann, 2002). The former type of text can be assumed to faithfully represent (aspects of) the personality, thoughts, or both, of the creator of that text. The latter type of text is generated for an instrumental reason, such as to persuade an audience, and may not reveal much about the speaker or author of that text. Hermann (1980, 2002) favored spontaneous remarks of decision makers for her content analyses, because her objective was to ascertain personality traits, and she expected spontaneous remarks to be far more representational than prepared speeches. Eshbaugh-Soha (2006), on the other hand, was interested in the instrumental use of language by presidents. For him, the prepared speeches of these decision makers were the more appropriate text. In other words, the text chosen for analysis should match the purposes of that analysis, just as the coding manual should be geared to the analytic purposes of the study.
Last, Babbie (2004) made a useful distinction between the unit of analysis and the unit of observation. If, for instance, you are interested in ascertaining the personality traits of a decision maker, then that decision maker is the unit of analysis—the entity about which you want to be able to make statements as a result of your investigation. To arrive at your conclusions about the individual decision maker, however, you may need to analyze multiple (and often a relatively large number) of spontaneous interview responses. Each of these interview responses is a unit of observation. Although a single interview response can be revealing, it is impossible to know whether it represents a typical statement of the subject under investigation unless it is compared with additional responses. Coding multiple units of observation permits the investigator to discern patterns and to make generalizable statements about the unit of analysis.
The previous section suggests that the use of human coders requires an assessment of the consistency of the coding decisions made by each human coder, as well as an evaluation of the congruity of the decisions made by different human coders. The former is generally referred to as the stability of the coding decisions, and the latter measures the reliability of the coding scheme.
Reliability requires that the coding scheme should lead different human coders to code the same text in the same way. That is, if the coding categories are sufficiently clear and explicit, trained human coders should exhibit little variation in the way they evaluate text. Whether human coders do indeed exhibit such agreement can be evaluated empirically. In doing so, it is important to compare the agreement on specific coding decisions rather than in the aggregate. For instance, when comparing the overall results of two coders, the data may look similar, but this similarity may evaporate when considering the individual coding decisions. If each coder made 100 coding decisions on one dimension and each assigned 50 of these to Category A, 30 to Category B, and the remaining 20 to Category C, their results look identical. However, when comparing Decision 1, Decision 2, Decision 3, and so on, it may become apparent that this overall congruity hides substantially different judgments regarding the discrete coding decisions. It is therefore recommended to analyze the similarity between individual coding decisions.
In addition, the calculation of an intercoder reliability score must take into account the role of chance. The coders must do better than agreement that results from chance in order for the similarity in their coding decisions to be meaningfully attributed to the clarity of the coding instructions. Both Krippendorff (1980) and Holsti (1969) provide detailed guidance for calculating intercoder reliability.
Well-designed and carefully implemented content analysis studies usually report an intercoder reliability statistic to demonstrate that the coding instrument is indeed sufficiently explicit to permit a high level of agreement between the coding decisions made by different human coders.
In addition, content analysis studies are often concerned with stability, which is defined as the consistency of the coder’s decisions across the texts she or he evaluates. Inconsistent decisions can result from coder fatigue but also from slight shifts in the implementation of a coding scheme between the first and last items coded. To evaluate the consistency of the coding decisions across texts and time, coders are sometimes asked to recode some of the text coded early in the project. The coding decisions made by a single coder at these two different times are then compared. If the individual coder makes largely the same decisions, the coding is judged to be stable. The calculation of the stability of the coding can be done using the same statistical tools used to determine the intercoder reliability score. The only difference is that stability measures the consistency of a single human coder, whereas the reliability score measures the consistency of the coding decisions across different human coders.
Content analysis predates the invention of computers. However, the increasing availability and current ubiquity of computers has revolutionized this methodology. Studies that would have required countless hours of meticulous attention to detail by several human coders can now be completed with much greater reliability and at much higher speed by computers.
Numerous software packages for content analysis have been developed. Some of these have been designed for very specific purposes (such as Diction), whereas other programs try to accommodate a variety of content analysis purposes (e.g., Atlas.ti, ProfilerPlus, and Wordstat). New programs enter the market on a regular basis, and older ones disappear. For this reason, this research paper does not include an overview of available programs.
When investigating software for content analysis, carefully evaluate whether the program suits the purposes for which it will be used. Each program has been developed with a particular purpose in mind and will excel at certain things and be less useful for other purposes. In other words, it is impossible to judge the merits of content analysis software in the abstract and without reference to the purposes for which it is being considered.
The speed with which computers can analyze large volumes of text is not the only advantage. Reliability essentially becomes a nonissue, because a computer program will analyze the same text in the same way no matter how often one asks it to analyze that text. This led West and Fuller (2001) to state that the “value of computer-assisted content analysis, particularly in terms of reliability, is difficult to overstate” (p. 91).
At the same time, it is important to note that computer-assisted content analysis depends for its validity on the careful design of the coding scheme. This remains the work of the investigator. A reliably executed computer-assisted content analysis cannot be better than the coding scheme it implements. Human coders are more likely to point out the flaws in the logic of the study’s design, whereas a computer will complete the coding task without question, even if it makes no substantive sense. Hence, it will remain important to obtain feedback on the draft of the coding scheme and to run pilot tests on small amounts of text to ensure that the coding scheme is well designed and appropriate for its purpose. Computers are extremely useful in the mechanical task of coding and can complete such tasks far more reliably than human coders, but the task of theoretically grounding the content analysis, evaluating whether the coding scheme tests what it claims to test, and deciding whether the coding categories meet the appropriate standards of clarity, explicitness, and validity will remain tasks that only the investigator can competently execute.
This research paper endeavors to provide a primer for the sort of research tasks for which content analysis is suited, as well as to suggest basic problems in the design of content analysis studies. The biggest drawback of content analysis has traditionally been that it was very time-consuming to complete large-scale studies. The advent of computer-assisted content analysis has given new life to this research methodology, and numerous software packages have been developed for this technique. Some of the initial software was suitable for only very specific coding tasks that mirrored the developer’s research purposes. More recently, there has been a greater emphasis on the development of software that can be adapted to a multitude of content analysis research designs. Atlas.ti, ProfilerPlus, and Wordstat are part of this new generation of software for content analysis.
One of the critiques of content analysis has long been that it tends to be atheoretical. That was true of some studies but does not adequately capture the more vexing problems that have plagued content analysis in political science. Content analysis is, in some instances, an end in itself. Studies that seek to analyze communicative content systematically, for instance, are examples of this form of content analysis. However, studies that endeavored to analyze the personality characteristics of leaders or the impact of the message often also stopped at analyzing text, when such studies in fact sought to make statements about something beyond the text. Studies focusing on the traits of leaders, for instance, have long claimed that leader personality influences the types of decisions that such individuals make. It is impossible to demonstrate this convincingly on the basis of the results of content analysis alone.
Recent content analysis studies have begun to use computer-assisted content analysis in combination with other variables. Since less time and effort is spent on the completion of the content analysis itself, researchers are more willing to combine the data derived from that analysis with additional variables. Content analysis has thus become a tool for generating data rather than an end in itself.
As computer-assisted content analysis has increasingly become the norm, this methodology has gained an expanded purpose in political science. Content analysis can now be used as a data-making tool that can yield quantitative indicators of aspects of political life that previously were deemed difficult or impossible to measure. For example, rather than claiming that certain personality traits create a disposition toward certain policy responses, investigators can now evaluate systematically whether this is the case. The results of content analysis can now be employed as variables in models that can more directly test the relationship between the traits of leaders and their actions, as well as establish more explicitly the relationship between speeches and their impact on political decisions.
Although there will remain cases where the analysis of the content of text is an end in itself, the more interesting research frontier for political science lies in using this methodology to systematically generate data. Combining this data with additional measures will permit analysts to evaluate the propositions that drew them to content analysis in the first place.
Bibliography:
- Babbie, E. (2004). The practice of social research (10th ed.). Belmont, CA: Wadsworth.
- Berelson, B. (1952). Content analysis in communication research. Glencoe, IL: Free Press.
- Breuning, M., Bredehoft, J., & Walton, E. (2005). Promise and performance: An evaluation of journals in international relations. International Studies Perspectives, 6(4), 447-461.
- Breuning, M., & Ishiyama, J. (1998). The rhetoric of nationalism: Rhetorical strategies of the Volksunie and Vlaams Blok in Belgium, 1991 1995. Political Communication, 15(1), 5-26.
- Bryman, A. (2004). Social science research methods (2nd ed.). Oxford, UK: Oxford University Press.
- Eshbaugh Soha, M. (2006). The president’s speeches: Beyond “going public.” Boulder, CO: Lynne Rienner.
- Hermann, M. G. (1980). Explaining foreign policy behavior using the personal characteristics of political leaders. International Studies Quarterly, 24, 7-46.
- Hermann, M. G. (2002). Assessing leadership style: A trait analysis. Hilliard, OH: Social Science Automation. Available at http://socialscience.net/docs/lta.pdf
- Holsti, O. (1969). Content analysis for the social sciences and humanities. Reading, MA: Addison Wesley.
- Krippendorff, K. (1980). Content analysis: An introduction to its methodology. Beverly Hills, CA: Sage.
- Neumann, I. B. (2008). Discourse analysis. In A. Klotz & D. Prakash (Eds.), Qualitative methods in international relations: A practical guide (pp. 61-77). Basingstoke, UK: Palgrave Macmillan.
- Walker, S. G., Schafer, M., & Young, M. D. (1998). Systematic procedures for operational code analysis: Measuring and modeling Jimmy Carter’s operational code. International Studies Quarterly, 42(1), 175-190.
- Weber, R. P. (1990). Basic content analysis (2nd ed.). Newbury Park, CA: Sage.
- West, M. D., & Fuller, L. K. (2001). Toward a typology and theoretical grounding for computer content analysis. In M. D. West (Ed.), Theory, method, and practice in computer content analysis (pp. 77-96). Westport, CT: Ablex.
- Winter, D. (2005). Measuring the motives of political actors at a distance. In J. Post (Ed.), The psychological assessment of political leaders (pp. 153-177). Ann Arbor: University of Michigan Press.
ORDER HIGH QUALITY CUSTOM PAPER

Research Paper Analysis: How to Analyze a Research Article + Example
Why might you need to analyze research? First of all, when you analyze a research article, you begin to understand your assigned reading better. It is also the first step toward learning how to write your own research articles and literature reviews. However, if you have never written a research paper before, it may be difficult for you to analyze one. After all, you may not know what criteria to use to evaluate it. But don’t panic! We will help you figure it out!
In this article, our team has explained how to analyze research papers quickly and effectively. At the end, you will also find a research analysis paper example to see how everything works in practice.
- 🔤 Research Analysis Definition
📊 How to Analyze a Research Article
✍️ how to write a research analysis.
- 📝 Analysis Example
- 🔎 More Examples
🔗 References
🔤 research paper analysis: what is it.
A research paper analysis is an academic writing assignment in which you analyze a scholarly article’s methodology, data, and findings. In essence, “to analyze” means to break something down into components and assess each of them individually and in relation to each other. The goal of an analysis is to gain a deeper understanding of a subject. So, when you analyze a research article, you dissect it into elements like data sources , research methods, and results and evaluate how they contribute to the study’s strengths and weaknesses.
📋 Research Analysis Format
A research analysis paper has a pretty straightforward structure. Check it out below!
Research articles usually include the following sections: introduction, methods, results, and discussion. In the following paragraphs, we will discuss how to analyze a scientific article with a focus on each of its parts.
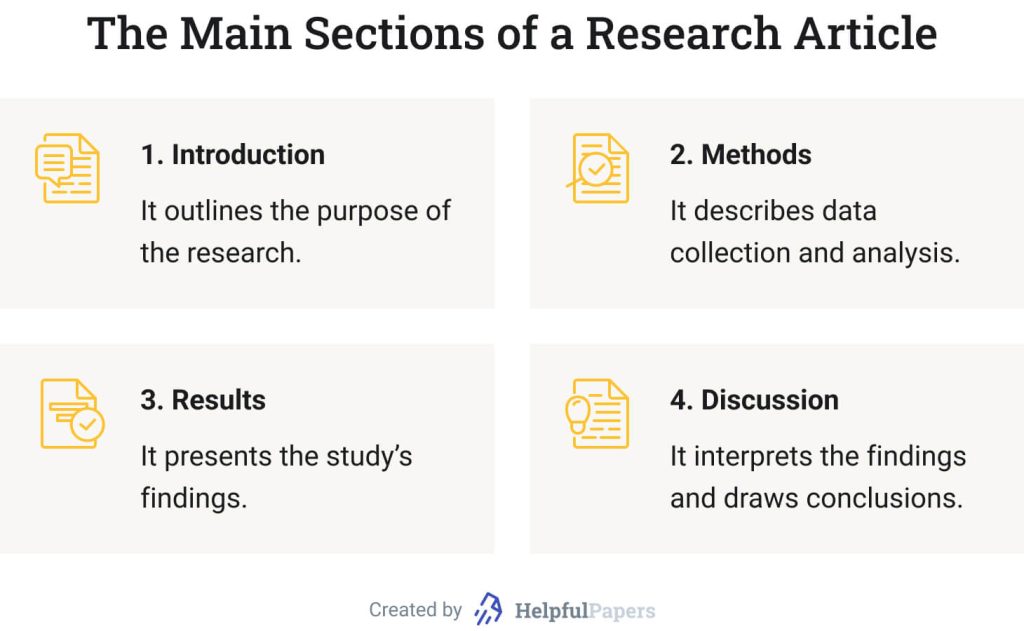
How to Analyze a Research Paper: Purpose
The purpose of the study is usually outlined in the introductory section of the article. Analyzing the research paper’s objectives is critical to establish the context for the rest of your analysis.
When analyzing the research aim, you should evaluate whether it was justified for the researchers to conduct the study. In other words, you should assess whether their research question was significant and whether it arose from existing literature on the topic.
Here are some questions that may help you analyze a research paper’s purpose:
- Why was the research carried out?
- What gaps does it try to fill, or what controversies to settle?
- How does the study contribute to its field?
- Do you agree with the author’s justification for approaching this particular question in this way?
How to Analyze a Paper: Methods
When analyzing the methodology section , you should indicate the study’s research design (qualitative, quantitative, or mixed) and methods used (for example, experiment, case study, correlational research, survey, etc.). After that, you should assess whether these methods suit the research purpose. In other words, do the chosen methods allow scholars to answer their research questions within the scope of their study?
For example, if scholars wanted to study US students’ average satisfaction with their higher education experience, they could conduct a quantitative survey . However, if they wanted to gain an in-depth understanding of the factors influencing US students’ satisfaction with higher education, qualitative interviews would be more appropriate.
When analyzing methods, you should also look at the research sample . Did the scholars use randomization to select study participants? Was the sample big enough for the results to be generalizable to a larger population?
You can also answer the following questions in your methodology analysis:
- Is the methodology valid? In other words, did the researchers use methods that accurately measure the variables of interest?
- Is the research methodology reliable? A research method is reliable if it can produce stable and consistent results under the same circumstances.
- Is the study biased in any way?
- What are the limitations of the chosen methodology?
How to Analyze Research Articles’ Results
You should start the analysis of the article results by carefully reading the tables, figures, and text. Check whether the findings correspond to the initial research purpose. See whether the results answered the author’s research questions or supported the hypotheses stated in the introduction.
To analyze the results section effectively, answer the following questions:
- What are the major findings of the study?
- Did the author present the results clearly and unambiguously?
- Are the findings statistically significant ?
- Does the author provide sufficient information on the validity and reliability of the results?
- Have you noticed any trends or patterns in the data that the author did not mention?
How to Analyze Research: Discussion
Finally, you should analyze the authors’ interpretation of results and its connection with research objectives. Examine what conclusions the authors drew from their study and whether these conclusions answer the original question.
You should also pay attention to how the authors used findings to support their conclusions. For example, you can reflect on why their findings support that particular inference and not another one. Moreover, more than one conclusion can sometimes be made based on the same set of results. If that’s the case with your article, you should analyze whether the authors addressed other interpretations of their findings .
Here are some useful questions you can use to analyze the discussion section:
- What findings did the authors use to support their conclusions?
- How do the researchers’ conclusions compare to other studies’ findings?
- How does this study contribute to its field?
- What future research directions do the authors suggest?
- What additional insights can you share regarding this article? For example, do you agree with the results? What other questions could the researchers have answered?
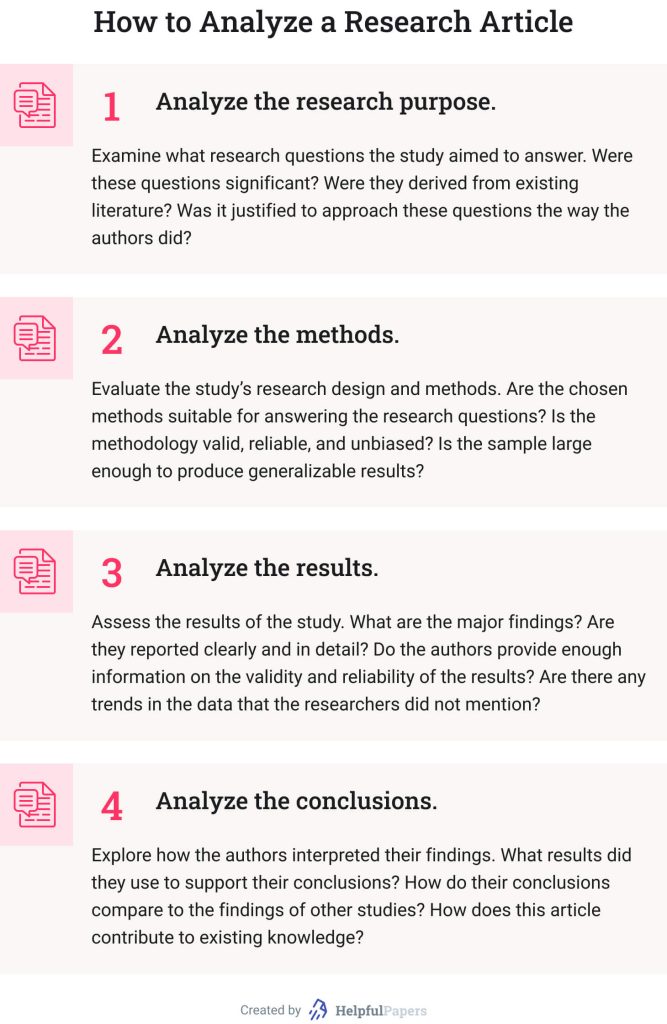
Now, you know how to analyze an article that presents research findings. However, it’s just a part of the work you have to do to complete your paper. So, it’s time to learn how to write research analysis! Check out the steps below!
1. Introduce the Article
As with most academic assignments, you should start your research article analysis with an introduction. Here’s what it should include:
- The article’s publication details . Specify the title of the scholarly work you are analyzing, its authors, and publication date. Remember to enclose the article’s title in quotation marks and write it in title case .
- The article’s main point . State what the paper is about. What did the authors study, and what was their major finding?
- Your thesis statement . End your introduction with a strong claim summarizing your evaluation of the article. Consider briefly outlining the research paper’s strengths, weaknesses, and significance in your thesis.
Keep your introduction brief. Save the word count for the “meat” of your paper — that is, for the analysis.
2. Summarize the Article
Now, you should write a brief and focused summary of the scientific article. It should be shorter than your analysis section and contain all the relevant details about the research paper.
Here’s what you should include in your summary:
- The research purpose . Briefly explain why the research was done. Identify the authors’ purpose and research questions or hypotheses .
- Methods and results . Summarize what happened in the study. State only facts, without the authors’ interpretations of them. Avoid using too many numbers and details; instead, include only the information that will help readers understand what happened.
- The authors’ conclusions . Outline what conclusions the researchers made from their study. In other words, describe how the authors explained the meaning of their findings.
If you need help summarizing an article, you can use our free summary generator .
3. Write Your Research Analysis
The analysis of the study is the most crucial part of this assignment type. Its key goal is to evaluate the article critically and demonstrate your understanding of it.
We’ve already covered how to analyze a research article in the section above. Here’s a quick recap:
- Analyze whether the study’s purpose is significant and relevant.
- Examine whether the chosen methodology allows for answering the research questions.
- Evaluate how the authors presented the results.
- Assess whether the authors’ conclusions are grounded in findings and answer the original research questions.
Although you should analyze the article critically, it doesn’t mean you only should criticize it. If the authors did a good job designing and conducting their study, be sure to explain why you think their work is well done. Also, it is a great idea to provide examples from the article to support your analysis.
4. Conclude Your Analysis of Research Paper
A conclusion is your chance to reflect on the study’s relevance and importance. Explain how the analyzed paper can contribute to the existing knowledge or lead to future research. Also, you need to summarize your thoughts on the article as a whole. Avoid making value judgments — saying that the paper is “good” or “bad.” Instead, use more descriptive words and phrases such as “This paper effectively showed…”
Need help writing a compelling conclusion? Try our free essay conclusion generator !
5. Revise and Proofread
Last but not least, you should carefully proofread your paper to find any punctuation, grammar, and spelling mistakes. Start by reading your work out loud to ensure that your sentences fit together and sound cohesive. Also, it can be helpful to ask your professor or peer to read your work and highlight possible weaknesses or typos.
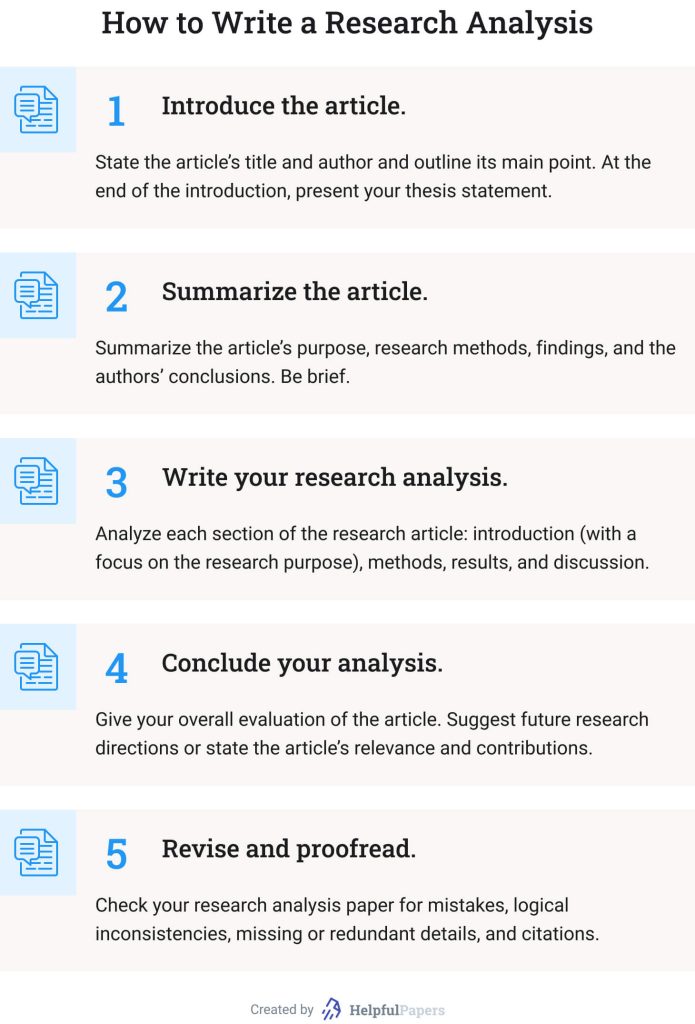
📝 Research Paper Analysis Example
We have prepared an analysis of a research paper example to show how everything works in practice.
No Homework Policy: Research Article Analysis Example
This paper aims to analyze the research article entitled “No Assignment: A Boon or a Bane?” by Cordova, Pagtulon-an, and Tan (2019). This study examined the effects of having and not having assignments on weekends on high school students’ performance and transmuted mean scores. This article effectively shows the value of homework for students, but larger studies are needed to support its findings.
Cordova et al. (2019) conducted a descriptive quantitative study using a sample of 115 Grade 11 students of the Central Mindanao University Laboratory High School in the Philippines. The sample was divided into two groups: the first received homework on weekends, while the second didn’t. The researchers compared students’ performance records made by teachers and found that students who received assignments performed better than their counterparts without homework.
The purpose of this study is highly relevant and justified as this research was conducted in response to the debates about the “No Homework Policy” in the Philippines. Although the descriptive research design used by the authors allows to answer the research question, the study could benefit from an experimental design. This way, the authors would have firm control over variables. Additionally, the study’s sample size was not large enough for the findings to be generalized to a larger population.
The study results are presented clearly, logically, and comprehensively and correspond to the research objectives. The researchers found that students’ mean grades decreased in the group without homework and increased in the group with homework. Based on these findings, the authors concluded that homework positively affected students’ performance. This conclusion is logical and grounded in data.
This research effectively showed the importance of homework for students’ performance. Yet, since the sample size was relatively small, larger studies are needed to ensure the authors’ conclusions can be generalized to a larger population.
🔎 More Research Analysis Paper Examples
Do you want another research analysis example? Check out the best analysis research paper samples below:
- Gracious Leadership Principles for Nurses: Article Analysis
- Effective Mental Health Interventions: Analysis of an Article
- Nursing Turnover: Article Analysis
- Nursing Practice Issue: Qualitative Research Article Analysis
- Quantitative Article Critique in Nursing
- LIVE Program: Quantitative Article Critique
- Evidence-Based Practice Beliefs and Implementation: Article Critique
- “Differential Effectiveness of Placebo Treatments”: Research Paper Analysis
- “Family-Based Childhood Obesity Prevention Interventions”: Analysis Research Paper Example
- “Childhood Obesity Risk in Overweight Mothers”: Article Analysis
- “Fostering Early Breast Cancer Detection” Article Analysis
- Lesson Planning for Diversity: Analysis of an Article
- Journal Article Review: Correlates of Physical Violence at School
- Space and the Atom: Article Analysis
- “Democracy and Collective Identity in the EU and the USA”: Article Analysis
- China’s Hegemonic Prospects: Article Review
- Article Analysis: Fear of Missing Out
- Article Analysis: “Perceptions of ADHD Among Diagnosed Children and Their Parents”
- Codependence, Narcissism, and Childhood Trauma: Analysis of the Article
- Relationship Between Work Intensity, Workaholism, Burnout, and MSC: Article Review
We hope that our article on research paper analysis has been helpful. If you liked it, please share this article with your friends!
- Analyzing Research Articles: A Guide for Readers and Writers | Sam Mathews
- Summary and Analysis of Scientific Research Articles | San José State University Writing Center
- Analyzing Scholarly Articles | Texas A&M University
- Article Analysis Assignment | University of Wisconsin-Madison
- How to Summarize a Research Article | University of Connecticut
- Critique/Review of Research Articles | University of Calgary
- Art of Reading a Journal Article: Methodically and Effectively | PubMed Central
- Write a Critical Review of a Scientific Journal Article | McLaughlin Library
- How to Read and Understand a Scientific Paper: A Guide for Non-scientists | LSE
- How to Analyze Journal Articles | Classroom
How to Write an Animal Testing Essay: Tips for Argumentative & Persuasive Papers
Descriptive essay topics: examples, outline, & more.
This paper is in the following e-collection/theme issue:
Published on 29.3.2024 in Vol 26 (2024)
#ProtectOurElders: Analysis of Tweets About Older Asian Americans and Anti-Asian Sentiments During the COVID-19 Pandemic
Authors of this article:

Original Paper
- Reuben Ng 1, 2 , PhD ;
- Nicole Indran 1 , BSocSci (Hons)
1 Lee Kuan Yew School of Public Policy, National University of Singapore, Singapore, Singapore
2 Lloyd's Register Foundation Institute for the Public Understanding of Risk, National University of Singapore, Singapore, Singapore
Corresponding Author:
Reuben Ng, PhD
Lee Kuan Yew School of Public Policy
National University of Singapore
469C Bukit Timah Road
Singapore, 259772
Phone: 65 66013967
Email: [email protected]
Background: A silver lining to the COVID-19 pandemic is that it cast a spotlight on a long-underserved group. The barrage of attacks against older Asian Americans during the crisis galvanized society into assisting them in various ways. On Twitter, now known as X, support for them coalesced around the hashtag #ProtectOurElders. To date, discourse surrounding older Asian Americans has escaped the attention of gerontologists—a gap we seek to fill. Our study serves as a reflection of the level of support that has been extended to older Asian Americans, even as it provides timely insights that will ultimately advance equity for them.
Objective: This study explores the kinds of discourse surrounding older Asian Americans during the COVID-19 crisis, specifically in relation to the surge in anti-Asian sentiments. The following questions guide this study: What types of discourse have emerged in relation to older adults in the Asian American community and the need to support them? How do age and race interact to shape these discourses? What are the implications of these discourses for older Asian Americans?
Methods: We retrieved tweets (N=6099) through 2 search queries. For the first query, we collated tweets with the hashtag #ProtectOurElders. For the second query, we collected tweets with an age-based term, for example, “elderly” or “old(er) adults(s)” and either the hashtag #StopAAPIHate or #StopAsianHate. Tweets were posted from January 1, 2020, to August 1, 2023. After applying the exclusion criteria, the final data set contained 994 tweets. Inductive and deductive approaches informed our qualitative content analysis.
Results: A total of 4 themes emerged, with 50.1% (498/994) of posts framing older Asian Americans as “vulnerable and in need of protection” (theme 1). Tweets in this theme either singled them out as a group in need of protection because of their vulnerable status or discussed initiatives aimed at safeguarding their well-being. Posts in theme 2 (309/994, 31%) positioned them as “heroic and resilient.” Relevant tweets celebrated older Asian Americans for displaying tremendous strength in the face of attack or described them as individuals not to be trifled with. Tweets in theme 3 (102/994, 10.2%) depicted them as “immigrants who have made selfless contributions and sacrifices.” Posts in this section referenced the immense sacrifices made by older Asian Americans as they migrated to the United States, as well as the systemic barriers they had to overcome. Posts in theme 4 (85/994, 8.5%) venerated older Asian Americans as “worthy of honor.”
Conclusions: The COVID-19 crisis had the unintended effect of garnering greater support for older Asian Americans. It is consequential that support be extended to this group not so much by virtue of their perceived vulnerability but more so in view of their boundless contributions and sacrifices.
Introduction
Not unlike other public health crises, the COVID-19 pandemic brought with it a disconcerting onslaught of racism and xenophobia [ 1 ]. The number of anti-Asian hate crimes in the United States quadrupled in 2021, escalating from the already significant uptick it experienced in 2020, when the COVID-19 outbreak was declared a global pandemic [ 2 ]. In the Asian American and Pacific Islanders (AAPI) community, those aged 60 years or older accounted for 7.3% of the 2808 self-reported incidents in 2020 [ 3 ]. Though not a particularly large figure, underreporting in this community is fairly common [ 4 ]. Moreover, older adults have reported being physically assaulted and having to deal with civil rights violations more than the general AAPI community [ 3 ]. When the crisis first emerged, older Asian Americans were beleaguered by increased economic insecurity [ 5 ] and poorer health outcomes [ 6 ] due to a confluence of structural inequities [ 5 ].
A silver lining to the COVID-19 pandemic is that it cast a spotlight on a long-underserved group. The barrage of attacks against older Asian Americans galvanized both individuals and organizations into assisting them in various ways, such as by distributing safety whistles and meal vouchers [ 7 ]. On Twitter, now known as X, support for them coalesced around the hashtag #ProtectOurElders [ 4 ]. The objective of this study is to explore the kinds of discourse surrounding older Asian Americans during the COVID-19 crisis, specifically in relation to the surge in anti-Asian sentiments.
Dating back to the nineteenth century, one of the most pervasive stereotypes of Asian Americans is that they are a high-achieving demographic [ 8 ]. While seemingly innocuous, this myth of them as a “model minority” has been criticized as highly problematic. Not only does it run counter to their lived realities—plenty of evidence has exposed the widespread inequalities confronted by various subgroups within the community [ 8 , 9 ]—it also delegitimizes their struggles and feeds the misconception that they require no assistance whatsoever [ 5 ].
Racial discrimination is well known to be a key social determinant of health [ 6 , 10 ]. Among Asian Americans in the United States, experiences of discrimination are linked to poorer mental health outcomes, including anxiety, depression, hypertension, and elevated blood pressure [ 10 ]. Racism may exacerbate health issues brought about by the aging process, such as the onset of chronic diseases or functional impairment [ 11 ], rendering older Asian Americans more susceptible to detrimental health outcomes.
Studies have indicated that social support has a positive impact on both the mental and physical health of older adults [ 12 ]. Social support likewise serves as a protective buffer against the negative effects of racial discrimination on one’s health [ 13 , 14 ]. The role of social support may be especially critical for Asian Americans. Although the Asian American populace includes a diverse array of ethnicities, cultures, and languages, collectivism appears to be a cultural orientation shared among many Asian American groups [ 15 ]. Evidence revealed that social support improved health outcomes among Asian Americans during the start of the pandemic, when anti-Asian sentiments were rampant [ 14 ].
It is widely acknowledged that in Asian societies, attitudes toward older adults are typically informed by values of respect and filial piety [ 11 , 16 ]. Old age bespeaks knowledge and wisdom, and younger people are expected to honor and respect their older counterparts [ 11 ]. Despite concerns that such values have eroded, there is evidence that they continue to resonate with Asian Americans [ 17 ]. One study concluded that Asian Americans are twice as likely as the general population to care for their parents [ 18 ]. Even so, ageism has been discovered to be pan-cultural [ 19 ]. A meta-analysis comparing Western and Eastern attitudes toward older adults revealed that Easterners actually harbored more negative views of older adults than Westerners [ 20 ]. In this analysis, Western countries included anglophone countries in the West such as Australia, Canada, the United Kingdom, and the United States, as well as Western European countries like Switzerland and France. Eastern countries covered countries in different regions of Asia, such as East Asia, South Asia, and Southeast Asia [ 20 ].
First proposed in 2002, the stereotype content model maintains that people stereotype others on the basis of warmth and competence [ 21 ]. The dimension of warmth includes qualities such as friendliness and sincerity, while the dimension of competence includes traits such as intelligence and skillfulness [ 21 ]. According to the stereotype content model, perceptions of social groups can be categorized into four clusters: (1) warm and competent, (2) incompetent and cold, (3) competent and cold, and (4) warm and incompetent. These 4 combinations of stereotypes produce distinct emotional responses among those who hold them. Groups stereotyped as warm and competent elicit admiration. Those evaluated as incompetent and cold elicit contempt. Groups stereotyped as competent and cold evoke envy. Those evaluated as warm but incompetent evoke pity [ 21 ].
A large body of work has evinced that older adults are generally stereotyped as warm but incompetent [ 21 ]. Although they elicit feelings of admiration occasionally, they predominantly evoke pity. Evidence attests to the universality of these stereotypes in both individualistic and collectivistic societies [ 19 ]. The evaluation of older adults as warm but lacking in competence may lend itself to benevolent ageism—a paternalistic form of prejudice founded on the assumption that older adults are helpless or pitiful [ 22 ]. Benevolent ageism has intensified over the course of the pandemic owing to recurring depictions of older adults as an at-risk group [ 23 ].
Asian Americans—older or otherwise—are one of the most underresearched ethnic groups in peer-reviewed literature [ 24 , 25 ]. In spite of the discomfiting rise in violence directed at them during the COVID-19 outbreak, discourse surrounding older adults from the Asian American community has escaped the attention of gerontologists. Most social media analyses conducted before and during the pandemic have focused on the discursive construction of the older population as a whole [ 26 - 28 ]. Other social media analyses have concentrated on the general Asian American population [ 29 - 31 ]. This study is therefore conceptually significant in that it is the first to dissect the content of tweets posted about older Asian Americans during the COVID-19 crisis.
At the heart of the concept of intersectionality is the notion that various social positions—such as race, age, gender, and socioeconomic status—interact to shape the types of biases one confronts [ 32 ]. From an intersectional standpoint, age and race may converge in ways that worsen the experience of discrimination for older Asian Americans [ 33 ]. In addition to being part of a racial group that faces more systemic challenges compared to White people, older Asian Americans also face age-related hurdles [ 34 ]. In terms of practical significance, this study serves as a reflection of the level of support being extended to older Asian Americans, even as it provides timely insights that will ultimately advance equity for them.
This study pivots around the following questions: What types of discourse have emerged in relation to older Asian Americans and the need to support them? How do age and race interact to shape these discourses? What are the implications of these discourses for older Asian Americans?
We retrieved the data using version 2 of Twitter’s application programming interface (API) [ 35 ], which was accessed through Twitter’s academic research product track [ 36 ]. Compared to what was achievable with the standard version 1.1 API, the version 2 API grants users a higher monthly tweet cap and access to more precise filters [ 37 ].
To build an extensive data set, we collected the tweets using 2 search queries. For both queries, “retweets” were excluded, and only English tweets posted from January 1, 2020, to August 1, 2023, were collated. We excluded retweets to avoid including duplicate content in the data set, which could skew the significance of particular topics. Tweets collected through the first query (n=1549) contained the hashtag #ProtectOurElders. For the second query (n=4550), we gathered tweets that met the following inclusion criteria: (1) contained either the hashtag #StopAAPIHate or #StopAsianHate; (2) included “elder,” “elderly,” “old(er) adult(s),” “old(er) people,” “old(er) person(s),” “senior(s),” “aged,” “old folk(s),” “grandparent(s),” “grandfather(s),” “grandmother(s),” “grandpa,” or “grandma.” The 2 queries yielded a total of 6099 tweets.
We removed posts that were (1) contextually irrelevant, that is, discussed content not pertaining to anti-Asian attacks, such as tweets related to getting vaccinated to protect older people, or tweets related to protecting older adults from cybercrime (n=1384); (2) repeated in the 2 queries (n=20); (3) incorrectly retrieved by the API, that is, they did not fulfill the inclusion criteria of either search query (n=258); and (4) informative, factual, or descriptive (eg, tweets that were newspaper headlines) or that brought up the older person in a tangential fashion (eg, tweets that mentioned older Asian Americans alongside several other groups; n=3443). After applying the aforementioned exclusion criteria, the data set consisted of 994 tweets. Figure 1 provides a flowchart of the data collection process.

Tweet Content Coding
Consistent with past research [ 27 , 38 - 41 ], the codebook was designed through both deductive and inductive modes of reasoning [ 42 ]. Analyses led by a directed or deductive approach begin with the identification of an initial set of codes based on previous literature [ 43 ]. Conversely, in inductive content analyses, codes are derived directly from the data [ 43 ]. We used both deductive and inductive approaches to make sure certain pertinent assumptions guided the analysis while also being aware that new categories would surface inductively [ 42 ].
To create a preliminary codebook, we first identified a set of categories based on previous literature regarding the perceptions of older adults in Asia [ 44 ]. The content analysis was subsequently conducted in several stages, with each tweet read twice by 2 researchers trained in gerontology to ensure familiarity with and immersion in the data [ 43 ]. The goal of the first reading was to ascertain the validity of the initial set of categories as well as to generate codes systematically across the whole data set. Each researcher modified the codebook independently until all variables were refined and clearly defined. During this first reading, a new category was added whenever a post featured a particular trait that could not be suitably coded into any of the existing categories and which was recurrent in the data. During the second reading, the 2 coders had frequent discussions where any discrepancies were reviewed and adjudicated to ensure rigor in the analysis. At this point, both coders discussed what the codes meant, confirmed the relevance of the codes to the research question, and identified areas of significant overlap to finalize the coding rubric.
The percentage agreement between the 2 raters was 92.5% with a weighted Cohen κ of 0.89 (P<.001), indicating high interrater reliability. A total of 4 themes emerged from the whole process. The frequency of each theme was identified after the analysis. As mentioned in past scholarship, categories in a content analysis need not be mutually exclusive, although they should be internally homogeneous (ie, coherent within themes) and externally heterogeneous (ie, distinct from each other) as far as possible [ 27 , 45 ].
Ethical Considerations
Ethical approval was not deemed necessary for this study, as all the data used were publicly available and anonymized.
Summary of Insights From Content Analysis of Tweets
A total of 4 themes emerged from our content analysis of 994 tweets. Half of the posts (498/994, 50.1%) were filed under the theme “vulnerable and in need of protection” (theme 1). Tweets in this theme either singled out older Asian Americans as a group in need of protection because of their vulnerable status or discussed initiatives aimed at safeguarding their well-being. The theme “heroic and resilient” (theme 2) was present in 31.1% (309/994) of the posts. Relevant tweets celebrated older Asian Americans for displaying tremendous strength in the face of attack or described them as individuals not to be trifled with. The theme “immigrants who have made selfless contributions and sacrifices” (theme 3) appeared in 10.2% (102/994) of the posts. Posts in this section referenced the immense sacrifices made by older Asian Americans as they migrated to the United States, as well as the systemic barriers they had to overcome. Theme 4 “worthy of honor” (85/994, 8.5%) consisted of tweets that venerated older Asian Americans. Textbox 1 provides a summary of the themes.
Vulnerable and in need of protection (498/994, 50.1%)
- “Isn't it so cowardly that they attack the elderly mostly? Not that violence is acceptable for any age, but to hurt the defenseless only means they got loose screws. #StopAsianHate”
- “Conducting walking patrols everyday to protect our elders and community #StopAAPIHate #HateisaVirus #StopAsianHate #SFChinatown #SafeNeighborhood #ProtectOurElders #TogetherWeCan”
Heroic and resilient (309/994, 31.1%)
- “Underestimating the terror wrought by old Chinese ladies with sticks was his first mistake #grannygoals #StopAsianHate”
- “Don't mess with Asian grandmas. But also sad this is happening. #StopAsianHate #StopAAPIHate”
Immigrants who have made selfless contributions and sacrifices (102/994, 10.2%)
- “Come to America they said..
It's the land of Opportunities they said...
Feeling so sad seeing this video 2 underage over privileged girls get to do this to a man ,a father ,a grandfather and not even have their identities revealed ...devastating
#MuhammadAnwar #StopAsianHate”
- “These are my grandparents. They came to America to build a new life. (That's my dad on the right wearing a tie.) My grandfather was a very well respected doctor in the Chinese community. America is built on the backbone of hard-working immigrants. #StopAsianHate”
Worthy of honor (85/994, 8.5%)
- “What's been shocking to me about these increased attacks on #AAPI is how often the elderly have been the focus. It’s such a shock because one thing that has been common amongst #AAPI culture is the reverence/respect of elders. #StopAAPIHate #StopAsianHate”
- “It really makes me weak and cry seeing videos of those elderly being hit and hurt. We, Asians, value and esteem our elderly. We even live with them in the same house, take care of them. I can't imagine how someone can simply push them. Just like that. #StopAsianHate”
Theme 1: Vulnerable and in Need of Protection
The vulnerability of older adults was a throughline in this category (498/994, 50.1%). Although concern was directed at the entire Asian American population, older adults were singled out as deserving of more sympathy because of their advanced age. Adjectives commonly used to frame them include “infirm,” “weak,” “defenseless,” and “powerless.” A person described them as lacking “the strength to even unclasp a grip.” Sympathy for older adults was magnified in view of other challenges they had been confronting since the outbreak of COVID-19. For instance, one poster expressed sorrow over how older Asian Americans had to grapple with the “fear of getting attacked” on top of “already [being] really afraid of COVID-19 because it disproportionately affects” them.
What made the act “especially egregious” in the eyes of many was the fact that assailants targeted older adults of all people. Users lambasted attackers for their “coward[ice],” asserting that they should have “picked on someone [their] own size” instead of attacking “people who can’t even defend themselves.” Several posters insisted that it was incumbent upon society to “be watchdogs” for older adults since they are more vulnerable.
A large number of tweets featured a call-to-action aimed at mobilizing members of the Twitter community to assist older Asian Americans. Fundraising campaigns were conducted to raise money for “alarms and pepper spray” for older Asian Americans. Others lobbied for donations to causes that deliver food to this group. The following tweet is one such example: “Wondering how you can support elderly Asians and show you will not tolerate #Asianhate? Join me in making a contribution to @heartofdinner, which brings food to elderly Asians in NYC so they can eat safely in their homes #StopAsianHateCrimes #StopAAPIHate.” The Twitter audience was also invited to escort older persons who walk alone: “United Peace Collaborative protects the #SF Chinatown community with daily walking patrols, providing protection & assistance to the elderly & residents. Please join us & volunteer!”
There were many tweets concerning the suite of initiatives aimed at supporting older Asian Americans. The Yellow Whistle—a campaign involving the distribution of free whistles for Asian Americans to signal danger in the event of an assault—was held up as one such example to “keep older Asian Americans safe.” Select community partners also received plaudits for their “wonderful work in distributing and training use of the alarms to” older persons.
Theme 2: Heroic and Resilient
Tweets in this theme (309/994, 31.1%) mainly revolved around a high-profile incident in San Francisco in which Xiao Zhen Xie, an older woman of Asian descent, put her assailant on a stretcher in an unexpected turn of events. She earned kudos from the Twitter community for “hold[ing] her ground,” “fighting back,” and sending him “to the hospital with his face bloodied.” Many saluted her for being “feisty,” “resilient,” and “[as] tough as nails,” dubbing her a “hero” who made them feel “#HonoredToBeAsian.” One user used the hashtag “#GrannyGoals,” quipping that the attacker made a “mistake” “underestimating the terror” that “old Chinese ladies” could wreak. Xiao Zhen Xie was also applauded for “refusing to be a statistic” as well as for defying the image of older adults as a group most expect “not to fight back.”
This episode involving Xiao Zhen Xie set in motion a series of tweets in which users warned others not to get on their grandparents’ bad side. A user cautioned that the incident was a lesson to everyone not to “mess with ahjummas, lolas, and all the elderly Asian women.” Another claimed that Asian grandmothers possess a special kind of “Asian grandma strength.” Some took the opportunity to underline the importance of not belittling older adults, with one in particular commenting on how his or her grandparents embodied grit and “toughness” because they “lived through war.”
Besides Xiao Zhen Xie, a few other older Asian Americans were celebrated for their resilience. A Filipina immigrant, Vilma Kari, was lauded for saying she “forgives” and “prays” for her attacker. A handful of tweets focused on a group of older Asian Americans who made the headlines for having filmed a music video in which they condemned the racially motivated acts of violence targeting their community.
Theme 3: Immigrants Who Have Made Selfless Contributions and Sacrifices
Members of the Twitter community frequently shared stories of their grandparents’ immigration (102/994, 10.2%). A common thread running through these posts was that their forefathers made immense sacrifices, uprooting themselves to move to the United States in order that their children might receive “the best education they can get” and “enjoy a “better future.” A user portrayed his or her grandmother as a “fighter” who “worked two to three jobs” while struggling to acculturate in a new society at a time when she knew “very little English.”
Attention was drawn to how the string of attacks against Asian Americans was ironic given the national ethos of the country commonly touted as the “American dream.” A few posters implied that labeling the United States as a “land of opportunity” was a misnomer: “Come to America,’ they said... ‘It’s the land of opportunities,’ they said...” A user said that the Asian “elderly did not escape communism” only to become a target of racism.
Tweets in this theme also discussed the burden of racism that older Asian Americans had endured before the COVID-19 pandemic. Users commented on their grandparents’ day-to-day experiences of racial discrimination. A handful were dismayed by how their grandparents were survivors of “prejudice and xenophobia” during World War II when they were forcibly relocated to Japanese internment camps. Others bemoaned that their older family members were “imprisoned for being the wrong-colored Americans.” One user deplored the fact that his or her grandfather “could not come to [the United States] because of his race” due to the Chinese Exclusion Act of 1882, a law that suspended Chinese immigration for 10 years and declared Chinese immigrants ineligible for naturalization. Another poster pinpointed how his or her grandfather felt compelled to dress in an “extremely patriotic” manner in order to camouflage his Asian identity and better assimilate into America.
Users considered older Asian Americans as foundational to the growth of America and foregrounded the need to acknowledge that “America is built on the backbone of hardworking immigrants,” who “made 90%” of what society has. Examples of contributions made by those of Asian ancestry include how they “oversaw” the construction of the transcontinental railroad in the “Old West” as well as their “service in the #442RCT (442nd Infantry Regiment)”—a highly decorated infantry regiment that mainly comprised second-generation American soldiers of Japanese descent who served in World War II. One user mentioned Chien-Shiung Wu, a groundbreaking Chinese American physicist whose scientific accomplishments were a core part of “U.S. WW II efforts” and that “helped win Nobel Prizes for Americans,” without which the “country would be so much worse off.” Artworks inspired by “hustling, elderly Asian folks” were also broadcasted under a hashtag that deified them as “#ChinatownGods.”
Several attempts were made to deconstruct the myth of the model minority. Individuals were aggrieved at how the looming specter of anti-Asian violence compounded the plight of older Asian Americans, who had already been dealt multiple blows during the COVID-19 crisis. These posters raised awareness of how many of them are in “precarious living situations” or “working in low-wage jobs.” Some pleaded for the Asian American community to be seen and understood, as captured in the following tweet: “See what’s happening to our elderly and community. Understand us. Understand why no matter how model of a minority we seem to be... we are just like you. #StopAsianHate #StopAAPIHate #StandWithAsians.”
Theme 4: Worthy of Honor
Many users (85/994, 8.5%) were outraged at how older adults appeared to be prime targets of violence against the Asian American community, perceiving these acts as a flagrant transgression of Asian cultural mores that “revere” them as “the most important people” in society. Some tweets exalted them as wellsprings of “wisdom” and “thoughtful guidance”—one user even likened them to “gold”—to “value and esteem.” Tweets in this theme also alluded to how deference to the older community was practically nonnegotiable in the Asian household. A poster tweeted, “No one should be assaulted, especially the elderly. I grew up respecting my elders. You never even argued with them ... They pass on wisdom.”
Values of collectivism were prized by certain users. These posters made reference to the notion of intergenerational reciprocity by stressing that younger people had an obligation to “protect” the older generation in return. The idea of solidarity was also raised. For instance, some viewed the attack of an older adult—related or otherwise—as an affront to the entire Asian community: “Many are saying ‘she could've been MY grandma.’ To that I say, she is ALL OUR GRANDMAS. Fight hate, love justice, stand with our elders always. #ForTheLoveOfLolas #StopAsianHate #StopAAPIHate #StopAsianHateCrimes.”
This study serves as a substantive first step in understanding discourses surrounding older Asian Americans. In our content analysis of tweets posted about the rash of attacks targeting them during the COVID-19 crisis, 4 main discourses surfaced. The first positioned them as “vulnerable and in need of protection” (theme 1). The second characterized them as “heroic and resilient” (theme 2). The third portrayed them as “immigrants who have made selfless contributions and sacrifices” (theme 3), and the fourth extolled them as “worthy of honor” (theme 4).
Our findings demonstrate an outpouring of support for the older Asian American community, which manifested itself in various local initiatives such as the distribution of safety whistles and the delivery of food. Scholars have drawn attention to how social support is particularly crucial for those in their later years [ 12 ] as well as those who experience racial discrimination [ 13 , 14 ]. The fact that older Asian Americans are finally being given support and assistance is therefore a step in the right direction.
However, even well-intentioned acts may be met with negative repercussions. In the wake of the COVID-19 crisis, older adults were reduced to a uniform group of at-risk individuals [ 46 ]. Assumptions of their vulnerability led to paternalistic behaviors, which denied them their autonomy [ 23 ]. Our results indicate that the rise in violence toward older Asian Americans sparked much-needed dialogue regarding their everyday struggles. Nevertheless, an unfortunate corollary is that this may have predisposed them to being recipients of benevolent prejudice on the basis of both age and race. Older Asian Americans may have been viewed as especially defenseless or vulnerable, perhaps more so than the general older population. This was made amply clear in the findings, where half of the tweets branded older Asian Americans as “weak” and “powerless.”
Notwithstanding concerns that Asian values of respect and filial piety have become irrelevant in the face of modernization [ 17 ], findings from themes 2-4 show emphatically that older adults retain their revered status, at least among some in the Asian American community. Tweets in theme 2 featured users enthusing over the way Xiao Zhen Xie held her ground when she was attacked in San Francisco, which led to deliberations on the strength and tenacity of older Asian women in general. Discourses of gratitude emerged in theme 3 as users ruminated over the sacrifices their forefathers had made in migrating to the United States, as well as the attendant systemic challenges they had to navigate. Posts in theme 4 indicate that users perceived the violence against older Asian Americans as a contravention of cultural norms, which emphasize the importance of honoring older adults. These provide a countervailing force to the various ageist tropes that came to the fore during the COVID-19 pandemic, such as the #BoomerRemover hashtag, which saw the lives of older people being discounted [ 27 , 28 ].
Theoretical Contribution and Implications
Findings from this study show that during the COVID-19 pandemic, age and race interfaced in complex ways to shape discourses on older Asian Americans. Specifically, our content analysis demonstrates that the stereotypes of warmth and incompetence, which are often thought to shape evaluations of older adults, cannot be applied indiscriminately to older Asian Americans as a subcategory of the older demographic. Theme 1, which positions older Asian Americans as vulnerable and in need of protection, does indeed align with traditional evaluations of older adults as warm and incompetent. However, the remaining themes celebrate older Asian Americans for their numerous contributions to society, the sacrifices they have made, and their unwavering resilience during the pandemic, all of which challenge the stereotype of incompetence under the stereotype content model. These findings add complexity to the commonly held notion of older adults as a pitiful social group by highlighting that older Asian Americans evoke not just pity but also admiration. The stereotype content model should therefore be expanded or modified in a way that accounts for attitudes toward older adults of different ethnicities.
Additionally, gerontological scholarship would benefit from a cross-cultural analysis of benevolent ageism. At present, little is known about how displays of benevolent ageism are affected by cultural norms of parental respect and filial piety and the extent to which these norms affect one’s perception of an older adult’s competence. Several studies have been conducted to make sense of ageism in different cultures [ 47 , 48 ], but there has been limited research on the cross-cultural differences in benevolent ageism specifically. The ways in which evaluations of older Asian Americans may be complicated by the deeply ingrained myth of the model minority as well as the pandemic-induced rise in anti-Asian hate are important avenues for future study.
This study has a number of implications for policy and practice. First, although care toward one’s parents or grandparents is not the prerogative of Asians [ 49 ], Asia’s adherence to collectivism nonetheless offers a useful learning point for the West. Many of the posters were Asian Americans, who held older adults in high regard, whether related or otherwise. Fostering a cultural emphasis on solidarity and interconnectedness in the West may promote respect not only for one’s parents but also for older adults outside of one’s family [ 44 ]. Second, ongoing efforts to reframe aging [ 50 ] could highlight the need to respect older adults, not in a way that advances their supremacy or absolves them from wrongdoing, but in a way that teaches society to view them as people whose experience may render them wise and worth learning from. Educators could also incorporate lessons on age-related stereotypes in schools to guard against the formation of ageist beliefs [ 51 ].
Third, current moves to redress the longstanding omission of Asian American history from national curricula [ 52 ] should ensure that students in every state are taught about the sacrifices, struggles, and contributions of older Asian Americans. Public campaigns could be organized as well to raise awareness of the aforementioned. This will help counter the myth of the model minority and get more people to acknowledge older Asian Americans as a significant part of America’s social fabric. Fourth, our findings underscore the need to reflect on the diversity of the older population in terms of socioeconomic status. Older adults—particularly those from the baby boomer generation—have been stereotyped as having made significant financial gains compared to their predecessors, at times even seen as stealing resources from the young [ 53 ]. However, as highlighted by some of the Twitter users as well as scholars, many older Asian Americans are in dire economic straits [ 5 ]. Rectifying the structural inequities that have contributed to their immiseration should hence be a key component of the agenda moving forward.
There are limitations inherent in this study. First, we acknowledge that Twitter users might not be representative of the wider population and that only publicly available tweets were included in the data set. Some of the users whose tweets were included in the study appeared to be Asian Americans, who are likely to be more passionate about supporting individuals in their community. Relatedly, as we did not collect information regarding users’ demographics—not all users publish demographic information, and there are certain limitations to using publicly provided demographic information on social media [ 54 ]—we could not contextualize the motivations of those whose tweets were included in the analysis. Ultimately, social support for older Asian Americans—whether from the Asian American community or society as a whole—has important implications for their well-being [ 14 ]. Subsequent research could focus on conducting interviews among individuals from different ethnic groups to tease out any differences in the level of support extended to older Asian Americans.
Second, we queried the hashtag #StopAAPIHate as a way to understand sentiments toward Asian Americans, even though the term “AAPI” refers to 2 different racial groups: Asian Americans and Pacific Islanders. As the tweets analyzed paid more attention to older Asian Americans, we were not able to offer insight into the types of discourses that emerged in relation to older Pacific Islanders. Future studies are needed to expound on such discourses. Third, it is vital to highlight that both the Asian American community and the older population are heterogeneous. The Asian American community encompasses numerous ethnicities, all with distinct languages, cultures, immigration histories, values, and beliefs [ 34 ]. The older demographic, too, is a diverse group composed of people with vastly different health trajectories [ 55 ]. Given the brevity of the tweets uploaded, we were unable to assess how discourses on older Asian Americans vary across different ethnicities. Finally, we collected only textual data, although tweets often contain visual elements such as photos, videos, and GIFs. This is a drawback that can be overcome in the future when multimodal techniques are developed to analyze both textual and visual content on Twitter.
Another direction for future inquiry involves an assessment of how discourses surrounding older Asian Americans have changed over time. The level of support shown to this group is likely to fluctuate over time, depending on the frequency at which anti-Asian attacks are reported in the news as well as other types of news being covered. Sentiment and narrative analyses [ 56 - 58 ] could be performed to glean such insights.
Even as older Asian Americans contended with a rise in racism alongside other struggles during the COVID-19 pandemic, our findings reveal that the crisis had the unintended effect of garnering greater support for this group. In the future, it is important that support be extended to older Asian Americans not so much by virtue of their perceived vulnerability but more so in view of their boundless contributions and sacrifices.
Acknowledgments
The authors would like to thank L Liu for preprocessing the data. We gratefully acknowledge support from the Social Science Research Council SSHR Fellowship (MOE2018-SSHR-004). The funder had no role in study design, data collection, analysis, writing, or the decision to publish this study.
Data Accessibility
Data are publicly available on Twitter [ 59 ].
Authors' Contributions
RN designed the study, developed the methodology, analyzed the data, wrote the paper, acquired the funding. RN and NI analyzed the data and wrote the paper.
Conflicts of Interest
None declared.
- Elias A, Ben J, Mansouri F, Paradies Y. Racism and nationalism during and beyond the COVID-19 pandemic. Ethn Racial Stud. 2021;44(5):783-793. [ CrossRef ]
- Yamauchi N. Anti-Asian hate crimes quadrupled in U.S. last year. Nikkei Asia. 2022. URL: https://asia.nikkei.com/Spotlight/Society/Anti-Asian-hate-crimes-quadrupled-in-U.S.-last-year [accessed 2022-02-16]
- Turton N. Stop AAPI Hate: new data on anti-Asian hate incidents against elderly and total national incidents in 2020. Stop AAPI Hate. 2021. URL: https://stopaapihate.org/wp-content/uploads/2021/04/Stop-AAPI-Hate-Press-Statement-Bay-Area-Elderly-Incidents-210209.pdf [accessed 2024-02-23]
- Huang J. How the #ProtectOurElders movement helped create a wave of first-time Asian American Activists. LAist. 2021. URL: https://laist.com/news/how-protectourelders-helped-created-a-wave-of-first-time-asian-american-activists [accessed 2022-04-03]
- Ma KPK, Bacong AM, Kwon SC, Yi SS, Ðoàn LN. The impact of structural inequities on older Asian Americans during COVID-19. Front Public Health. 2021;9:690014. [ FREE Full text ] [ CrossRef ] [ Medline ]
- Chen JA, Zhang E, Liu CH. Potential impact of COVID-19-related racial discrimination on the health of Asian Americans. Am J Public Health. 2020;110(11):1624-1627. [ CrossRef ] [ Medline ]
- Lee E. Asian American community rallies to support elders. AARP. 2021. URL: https://www.aarp.org/home-family/friends-family/info-2021/asian-american-support-communities.html [accessed 2022-02-16]
- Yi V, Museus S. Model minority myth. In: Smith AD, Hou X, Stone J, Dennis RM, Rizova P, editors. The Wiley Blackwell Encyclopedia of Race, Ethnicity, and Nationalism. Oxford, UK. John Wiley & Sons, Ltd; 2015.
- Li G, Wang L. Model Minority Myth Revisited: An Interdisciplinary Approach to Demystifying Asian American Educational Experiences. Charlotte, NC. Information Age Publishing; 2008.
- Paradies Y, Ben J, Denson N, Elias A, Priest N, Pieterse A, et al. Racism as a determinant of health: a systematic review and meta-analysis. PLoS One. 2015;10(9):e0138511. [ FREE Full text ] [ CrossRef ] [ Medline ]
- Min J, Moon A. Older Asian Americans. In: Berkman B, D'Ambruoso S, editors. Handbook of Social Work in Health and Aging. New York, NY. Oxford University Press; 2006.
- Antonucci TC, Ajrouch KJ, Birditt KS. The convoy model: explaining social relations from a multidisciplinary perspective. Gerontologist. 2014;54(1):82-92. [ FREE Full text ] [ CrossRef ] [ Medline ]
- Ajrouch KJ, Reisine S, Lim S, Sohn W, Ismail A. Perceived everyday discrimination and psychological distress: does social support matter? Ethn Health. 2010;15(4):417-434. [ FREE Full text ] [ CrossRef ] [ Medline ]
- Lee S, Waters SF. Asians and Asian Americans’ experiences of racial discrimination during the COVID-19 pandemic: impacts on health outcomes and the buffering role of social support. Stig Health. Feb 2021;6(1):70-78. [ CrossRef ]
- Markus HR, Kitayama S. The cultural construction of self and emotion: implications for social behavior. In: Emotion and Culture: Empirical Studies of Mutual Influence. Washington, DC. American Psychological Association; 1994;89-130.
- Ingersoll-Dayton B, Saengtienchai C. Respect for the elderly in Asia: stability and change. Int J Aging Hum Dev. 1999;48(2):113-130. [ CrossRef ] [ Medline ]
- Harrington B. "It's more us helping them instead of them helping us": how class disadvantage motivates Asian American college students to help their parents. J Fam Issues. 2022;44(7):1773-1795. [ CrossRef ]
- Montenegro X. Caregiving among Asian Americans and Pacific Islanders age 50+. AARP Research. 2014. URL: https://www.aarp.org/pri/topics/ltss/family-caregiving/caregiving-asian-americans-pacific-islanders/ [accessed 2024-02-23]
- Cuddy AJC, Norton MI, Fiske ST. This old stereotype: the pervasiveness and persistence of the elderly stereotype. J Social Issues. 2005;61(2):267-285. [ CrossRef ]
- North MS, Fiske ST. Modern attitudes toward older adults in the aging world: a cross-cultural meta-analysis. Psychol Bull. 2015;141(5):993-1021. [ CrossRef ] [ Medline ]
- Fiske ST, Cuddy AJC, Glick P, Xu J. A model of (often mixed) stereotype content: competence and warmth respectively follow from perceived status and competition. J Pers Soc Psychol. 2002;82(6):878-902. [ CrossRef ]
- Cary LA, Chasteen AL, Remedios J. The ambivalent ageism scale: developing and validating a scale to measure benevolent and hostile ageism. Gerontologist. 2017;57(2):e27-e36. [ FREE Full text ] [ CrossRef ] [ Medline ]
- Vervaecke D, Meisner B. Caremongering and assumptions of need: the spread of compassionate ageism during COVID-19. Gerontologist. 2021;61(2):159-165. [ FREE Full text ] [ CrossRef ] [ Medline ]
- Yi SS. Taking action to improve Asian American Health. Am J Public Health. 2020;110(4):435-437. [ CrossRef ] [ Medline ]
- Ðoàn LN, Takata Y, Sakuma KLK, Irvin VL. Trends in clinical research including Asian American, Native Hawaiian, and Pacific Islander participants funded by the US National Institutes of Health, 1992 to 2018. JAMA Netw Open. 2019;2(7):e197432. [ FREE Full text ] [ CrossRef ] [ Medline ]
- Levy BR, Chung PH, Bedford T, Navrazhina K. Facebook as a site for negative age stereotypes. Gerontologist. 2014;54(2):172-176. [ FREE Full text ] [ CrossRef ] [ Medline ]
- Sipocz D, Freeman JD, Elton J. "A toxic trend?": generational conflict and connectivity in Twitter discourse under the #BoomerRemover hashtag. Gerontologist. 2021;61(2):166-175. [ FREE Full text ] [ CrossRef ] [ Medline ]
- Skipper AD, Rose DJ. #BoomerRemover: COVID-19, ageism, and the intergenerational twitter response. J Aging Stud. 2021;57:100929. [ FREE Full text ] [ CrossRef ] [ Medline ]
- Hswen Y, Xu X, Hing A, Hawkins JB, Brownstein JS, Gee GC. Association of "#covid19" versus "#chinesevirus" with Anti-Asian sentiments on Twitter: march 9-23, 2020. Am J Public Health. 2021;111(5):956-964. [ FREE Full text ] [ CrossRef ] [ Medline ]
- Nguyen TT, Criss S, Dwivedi P, Huang D, Keralis J, Hsu E, et al. Exploring U.S. shifts in Anti-Asian sentiment with the emergence of COVID-19. Int J Environ Res Public Health. 2020;17(19):7032. [ FREE Full text ] [ CrossRef ] [ Medline ]
- Darling-Hammond S, Michaels EK, Allen AM, Chae DH, Thomas MD, Nguyen TT, et al. After "The China Virus" went viral: racially charged coronavirus coverage and trends in bias against Asian Americans. Health Educ Behav. 2020;47(6):870-879. [ FREE Full text ] [ CrossRef ] [ Medline ]
- Krekula C, Nikander P, Wilińska M. Multiple marginalizations based on age: gendered ageism and beyond. In: Ayalon L, Tesch-Römer C, editors. Contemporary Perspectives on Ageism. Cham, Switzerland. Springer Open; 2018;33-50.
- Gutterman AS. Ageism, race and ethnicity. SSRN Journal. Preprint posted online on December 8 2021. 2021. [ FREE Full text ] [ CrossRef ]
- Kim G, Wang SY, Park S, Yun SW. Mental health of Asian American older adults: contemporary issues and future directions. Innov Aging. 2020;4(5):igaa037. [ FREE Full text ] [ CrossRef ] [ Medline ]
- Twitter API v2: early access. Twitter. 2021. URL: https://developer.twitter.com/en/docs/twitter-api/early-access [accessed 2021-10-13]
- Tornes A, Trujillo L. Enabling the future of academic research with the Twitter API. Twitter Developer Platform Blog. 2021. URL: https://blog.twitter.com/developer/en_us/topics/tools/2021/enabling-the-future-of-academic-research-with-the-twitter-api [accessed 2021-10-13]
- Barrie C, Ho JC. academictwitteR: an R package to access the Twitter Academic Research Product Track v2 API endpoint. JOSS. 2021;6(62):3272. [ FREE Full text ] [ CrossRef ]
- Ng R, Indran N. Does age matter? Tweets about gerontocracy in the United States. J Gerontol B Psychol Sci Soc Sci. 2023;78(11):1870-1878. [ CrossRef ] [ Medline ]
- Ng R, Indran N. Innovations for an aging society through the lens of patent data. Gerontologist. 2024;64(2). [ FREE Full text ] [ CrossRef ] [ Medline ]
- Ng R, Indran N. Not too old for TikTok: how older adults are reframing aging. Gerontologist. 2022;62(8):1207-1216. [ FREE Full text ] [ CrossRef ] [ Medline ]
- Ng R, Indran N. Age advocacy on Twitter over 12 years. Gerontologist. 2024;64(1). [ CrossRef ] [ Medline ]
- Armat MR, Assarroudi A, Rad M, Sharifi H, Heydari A. Inductive and deductive: ambiguous labels in qualitative content analysis. TQR. 2018;23(1):219-221. [ FREE Full text ] [ CrossRef ]
- Hsieh HF, Shannon SE. Three approaches to qualitative content analysis. Qual Health Res. 2005;15(9):1277-1288. [ CrossRef ] [ Medline ]
- Hwang KK. Filial piety and loyalty: two types of social identification in Confucianism. Asian J of Social Psycho. 2002;2(1):163-183. [ CrossRef ]
- Bengtsson M. How to plan and perform a qualitative study using content analysis. NursingPlus Open. 2016;2:8-14. [ FREE Full text ] [ CrossRef ]
- Ayalon L. There is nothing new under the sun: ageism and intergenerational tension in the age of the COVID-19 outbreak. Int Psychogeriatr. 2020;32(10):1221-1224. [ FREE Full text ] [ CrossRef ] [ Medline ]
- Ng R, Lim-Soh JW. Ageism linked to culture, not demographics: evidence from an 8-billion-word corpus across 20 countries. J Gerontol B Psychol Sci Soc Sci. 2021;76(9):1791-1798. [ FREE Full text ] [ CrossRef ] [ Medline ]
- Löckenhoff CE, De Fruyt F, Terracciano A, McCrae RR, De Bolle M, Costa PT, et al. Perceptions of aging across 26 cultures and their culture-level associates. Psychol Aging. 2009;24(4):941-954. [ FREE Full text ] [ CrossRef ] [ Medline ]
- Lim AJ, Lau CYH, Cheng CY. Applying the Dual Filial Piety Model in the United States: a comparison of filial piety between Asian Americans and Caucasian Americans. Front Psychol. 2021;12:786609. [ FREE Full text ] [ CrossRef ] [ Medline ]
- Sweetland J, Volmert A, O'Neil M. Finding the frame: an empirical approach to reframing aging and ageism. FrameWorks Institute. Washington, DC.; 2017. URL: https://www.frameworksinstitute.org/wp-content/uploads/2020/05/aging_research_report_final_2017.pdf [accessed 2024-02-23]
- Russell ER, Thériault ÉR, Colibaba A. Facilitating age-conscious student development through lecture-based courses on aging. Can J Aging. 2022;41(2):283-293. [ FREE Full text ] [ CrossRef ] [ Medline ]
- Chavez N. New Jersey becomes second state to require Asian American history to be taught in schools. CNN. 2022. URL: https://www.cnn.com/2022/01/18/us/new-jersey-schools-asian-american-history/index.html [accessed 2022-04-11]
- Ng R, Indran N. Hostility toward baby Boomers on TikTok. Gerontologist. 2022;62(8):1196-1206. [ FREE Full text ] [ CrossRef ] [ Medline ]
- Sloan L. Who Tweets in the United Kingdom? Profiling the Twitter population using the British Social Attitudes Survey 2015. Soc Media Soc. 2017;3(1):205630511769898. [ FREE Full text ] [ CrossRef ]
- Diehl M, Smyer MA, Mehrotra CM. Optimizing aging: a call for a new narrative. Am Psychol. 2020;75(4):577-589. [ FREE Full text ] [ CrossRef ] [ Medline ]
- Ng R, Indran N, Suarez P. Communicating risk perceptions through Batik art. JAMA. 2023;330(9):790-791. [ CrossRef ] [ Medline ]
- Ng R, Indran N. Reframing aging: foregrounding familial and occupational roles of older adults is linked to decreased ageism over two centuries. J Aging Soc Policy. 2023.:1-18. [ CrossRef ] [ Medline ]
- Ng R, Indran N. Impact of old age on an occupation's image over 210 years: an age premium for doctors, lawyers, and soldiers. J Appl Gerontol. 2023;42(6):1345-1355. [ CrossRef ] [ Medline ]
- Twitter. 2021. URL: https://twitter.com/ [accessed 2021-10-13]
Abbreviations
Edited by A Mavragani; submitted 19.01.23; peer-reviewed by A Atalay, A Bacong; comments to author 22.02.23; revised version received 12.03.23; accepted 14.09.23; published 29.03.24.
©Reuben Ng, Nicole Indran. Originally published in the Journal of Medical Internet Research (https://www.jmir.org), 29.03.2024.
This is an open-access article distributed under the terms of the Creative Commons Attribution License (https://creativecommons.org/licenses/by/4.0/), which permits unrestricted use, distribution, and reproduction in any medium, provided the original work, first published in the Journal of Medical Internet Research, is properly cited. The complete bibliographic information, a link to the original publication on https://www.jmir.org/, as well as this copyright and license information must be included.

IMAGES
VIDEO
COMMENTS
Content analysis is a research method used to identify patterns in recorded communication. To conduct content analysis, you systematically collect data from a set of texts, which can be written, oral, or visual: Books, newspapers and magazines. Speeches and interviews. Web content and social media posts. Photographs and films.
Content analysis is a research tool used to determine the presence of certain words, themes, or concepts within some given qualitative data (i.e. text). ... questions, field research notes, conversations, or literally any occurrence of communicative language (such as books, essays, discussions, newspaper headlines, speeches, media, historical ...
Step 1: Select the content you will analyse. Based on your research question, choose the texts that you will analyse. You need to decide: The medium (e.g., newspapers, speeches, or websites) and genre (e.g., opinion pieces, political campaign speeches, or marketing copy)
Content analysis is the study of recorded human. communications such as dairy entries, books, newspaper, video s, text messages, tweets, Facebook updates etc. Being the scientific study of the ...
Content analysis is a research method used to analyze and interpret the characteristics of various forms of communication, such as text, images, or audio. It involves systematically analyzing the content of these materials, identifying patterns, themes, and other relevant features, and drawing inferences or conclusions based on the findings.
A common starting point for qualitative content analysis is often transcribed interview texts. The objective in qualitative content analysis is to systematically transform a large amount of text into a highly organised and concise summary of key results. Analysis of the raw data from verbatim transcribed interviews to form categories or themes ...
Content analysis is a qualitative analysis method that focuses on recorded human artefacts such as manuscripts, voice recordings and journals. Content analysis investigates these written, spoken and visual artefacts without explicitly extracting data from participants - this is called unobtrusive research. In other words, with content ...
Abstract. This paper describes the research process - from planning to presentation, with the emphasis on credibility throughout the whole process - when the methodology of qualitative content analysis is chosen in a qualitative study. The groundwork for the credibility initiates when the planning of the study begins.
content analysis as well as the advantages and disadvantages of using content analysis as a research methodology. Examples: View examples of real and hypothetical studies that use content analysis. Annotated Bibliography: Complete list of resources used in this guide and beyond. An Introduction to Content Analysis
They include making a great amount of money, being charitable, being a law-abiding citizen, making a good marriage and raising a large family. To get there, the cartoon suggests making a large amount of money (i.e. money features both as an end and a means), using force, and working hard.
Abstract. Content analysis is a highly fl exible research method that has been. widely used in library and infor mation science (LIS) studies with. varying research goals and objectives. The ...
Content analysis is a research method and type of textual analysis that analyzes the meanings of content, which could take the form of textual, visual, aural, and otherwise multimodal texts.. Generally, a content analysis will seek meanings and relationships of certain words and concepts within the text or corpus of texts, and generate thematic data that reveals deeper insights into the text ...
Here are a few insightful example using our text with 7 words: 7 word strings, inductive word frequency, content analysis. Perhaps more insightfully, here is a list of 5 word combinations, which are much more common: 5 word strings, inductive word frequency, content analysis. The downside to these tools is that you cannot find 2- and 1-word ...
Content analysis is a research method that can identify patterns in recorded communications. By systematically collecting data from different types of texts, such as written documents, speeches, web content or visual media, researchers can then discover valuable information about the goals, messages and effects of communication.
According to Gheyle and Jacobs (2017), content analysis is one of the research methods that, in short, could be defined as trying to determine the meaning behind textual context. It is how a ...
For this research, I used a mixed-method approach approved by an Institutional Review Board (IRB) to explore Barbies capability to be a role model based on the characters in her movies and how the movies have changed over time. This will be a content analysis research designed to determine the presence of themes in the movies.
For many researchers unfamiliar with qualitative research, determining how to conduct qualitative analyses is often quite challenging. Part of this challenge is due to the seemingly limitless approaches that a qualitative researcher might leverage, as well as simply learning to think like a qualitative researcher when analyzing data. From framework analysis (Ritchie & Spencer, 1994) to content ...
Definition: Research Paper is a written document that presents the author's original research, analysis, and interpretation of a specific topic or issue. It is typically based on Empirical Evidence, and may involve qualitative or quantitative research methods, or a combination of both. The purpose of a research paper is to contribute new ...
They grapple with qualitative research terms and concepts, for example; differences between meaning units, codes, categories and themes, and regarding increasing levels of abstraction from raw data to categories or themes. ... Content analysis, as in all qualitative analysis, is a reflective process. There is no "step 1, 2, 3, done!" linear ...
Simplistic Use of a Research Method . Score of 3 . Ineffectual Argument for a New Understanding . Score of 4 . Well-Supported, Articulate Argument Conveying a New Understanding . Score of 5 . Rich Analysis of a New Understanding Addressing a Gap in the Research Base . Presents an overly broad topic of inquiry. Presents a topic of inquiry with
As already mentioned, content analysis generally refers to quantitative assessment of various aspects of text. This research paper places its emphasis on such analysis. As outlined previously, content analysis is defined by the quest to analyze text in a manner that is systematic, valid, and replicable.
Save the word count for the "meat" of your paper — that is, for the analysis. 2. Summarize the Article. Now, you should write a brief and focused summary of the scientific article. It should be shorter than your analysis section and contain all the relevant details about the research paper.
Background: A silver lining to the COVID-19 pandemic is that it cast a spotlight on a long-underserved group. The barrage of attacks against older Asian Americans during the crisis galvanized society into assisting them in various ways. On Twitter, now known as X, support for them coalesced around the hashtag #ProtectOurElders. To date, discourse surrounding older Asian Americans has escaped ...